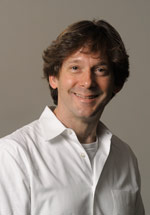
Mark A. Hasegawa-Johnson
(he/him/his)
Professor
Primary Affiliation
Biologically Informed Artificial IntelligenceAffiliations
Status Full-time Faculty
Home Department of Electrical and Computer Engineering
Phone 333-0925
Email jhasegaw@illinois.edu
Address 2011 Beckman Institute, 405 North Mathews Avenue
-
Biography
Mark Hasegawa-Johnson is a professor in the University of Illinois department of Electrical and Computer Engineering and a full-time faculty member in the Artificial Intelligence group at the Beckman Institute.
Education
Ph.D., Massachusetts of Technology, 1996
-
Honors
2023: Fellow of the International Speech Communication Association for contributions to knowledge-constrained signal generation
2020: Fellow of the IEEE, for contributions to speech processing of under-resourced languages
2011: Fellow of the Acoustical Society of America, for contributions to vocal tract and speech modeling
2009: Senior Member of the Association for Computing Machinery
2004: Member, Articulograph International Steering Committee; CLSP Workshop leader, "Landmark-Based Speech Recognition”, Invited paper
2004: NAACL workshop on Linguistic and Higher-Level Knowledge Sources in Speech Recognition and Understanding
2003: List of faculty rated as excellent by their students
2002: NSF CAREER award
1998: NIH National Research Service Award
-
Research
Research Interests
Acoustic phonetics
Audio signal processing and speech recognition
Speech and auditory physiology
Research Areas
Acoustics
Adaptive signal processing
Biomedical imaging
Computer vision and pattern recognition
Image, video, and multimedia processing and compression
Machine learning
Machine learning and pattern recognition
Natural language processing
Random processes
Robotics and motion planning
Signal detection and estimation
Signal Processing
Speech recognition and processing
Hasegawa-Johnson has been on the faculty at the University of Illinois since 1999. His research addresses automatic speech recognition with a focus on the mathematization of linguistic concepts. His group has developed mathematical models of concepts from linguistics including a rudimentary model of pre-conscious speech perception (the landmark-based speech recognizer), a model that interprets pronunciation variability by figuring out how the talker planned his or her speech movements (tracking of tract variables from acoustics, and of gestures from tract variables), and a model that uses the stress and rhythm of natural language (prosody) to disambiguate confusable sentences. Applications of his research include:
Speech recognition for talkers with cerebral palsy. The automatic system, suitably constrained, outperforms a human listener.
Provably correct unsupervised ASR, or ASR that can be trained using speech that has no associated text transcripts.
Equal Accuracy Ratio regularization: Methods that reduce the error rate gaps caused by gender, race, dialect, age, education, disability and/or socioeconomic class.
Automatic analysis of the social interactions between infant, father, mother, and older sibling during the first eighteen months of life.
Hasegawa-Johnson is currently Senior Area Editor of the journal IEEE Transactions on Audio, Speech and Language and a member of the ISCA Diversity Committee. He has published 308 peer-reviewed journal articles, patents, and conference papers in the general area of automatic speech analysis, including machine learning models of articulatory and acoustic phonetics, prosody, dysarthria, non-speech acoustic events, audio source separation, and under-resourced languages.
-
2016
- Chen, W.; Hasegawa-Johnson, M.; Chen, N. F., Mismatched Crowdsourcing Based Language Perception for under-Resourced Languages. Procedia Computer Science 2016, 81, 23-29, DOI:10.1016/j.procs.2016.04.025.
- Kong, X.; Jyothi, P.; Hasegawa-Johnson, M., Performance Improvement of Probabilistic Transcriptions with Language-Specific Constraints. Procedia Computer Science 2016, 81, 30-36, DOI:10.1016/j.procs.2016.04.026.
- Livescu, K.; Rudzicz, F.; Fosler-Lussier, E.; Hasegawa-Johnson, M.; Bilmes, J., Speech Production in Speech Technologies: Introduction to the CSL Special Issue. Computer Speech and Language 2016, 36, 165-172.
2015
- Hasegawa-Johnson, M.; Cole, J.; Jyothi, P.; Varshney, L. R., Models of Dataset Size, Question Design, and Cross-Language Speech Perception for Speech Crowdsourcing Applications. Laboratory Phonology 2015, 6, (3-4), 381-432.
- Huang, P. S.; Kim, M.; Hasegawa-Johnson, M.; Smaragdis, P., Joint Optimization of Masks and Deep Recurrent Neural Networks for Monaural Source Separation. IEEE-ACM Transactions on Audio Speech and Language Processing 2015, 23, (12), 2136-2147.
2014
- Chen, A.; Hasegawa-Johnson, M. A., Mixed Stereo Audio Classification Using a Stereo-Input Mixed-to-Panned Level Feature. IEEE-ACM Transactions on Audio Speech and Language  Processing 2014, 22, (12), 2025-2033, DOI:10.1109/Taslp.2014.2359628.
- Huang P.-S.; Kim, M.; Hasegawa-Johnson, M.; Smaragdis, P., Singing-Voice Separation from Monaural Recordings Using Deep Recurrent Neural Networks, Proceedings of the International Symposium of Music Information Retrieval, 2014, Taipei, Taiwan.
- Huang, P. S.; Kim, M.; Hasegawa-Johnson, M.; Smaragdis, P., Deep Learning for Monaural Speech Separation. IEEE International Conference on Acoustics, Speech and Signal Processing (ICASSP) 2014, Florence, Italy.
- Jyothi, P.; Cole, J.; Hasegawa-Johnson, M.; Puri, V., An Investigation of Prosody in Hindi Narrative Speech, Proceedings of Speech Prosody 2014, Volume 7. Dublin, Ireland.
- Khasanova, A.; Cole, J.; Hasegawa-Johnson, M., Detecting Articulatory Compensation in Acoustic Data through Linear Regression Modeling, Proceedings of Interspeech 2014, Singapore.
- Kim, K.; Lin, K. H.; Walther, D. B.; Hasegawa-Johnson, M. A.; Huang, T. S., Automatic Detection of Auditory Salience with Optimized Linear Filters Derived from Human Annotation. Pattern Recognition Letters 2014, 38, 78-85, DOI: 10.1016/j.patrec.2013.11.010.
2013
- Bharadwaj, S.; Hasegawa-Johnson, M.; Ajmera, J.; Deshmukh, O.; Verma, A.; Sparse Hidden Markov Models for Purer Clusters, In 2013 IEEE International Conference on Acoustics, Speech and Signal Processing. IEEE, New York, 2013, 3098-3102.
- Huang, P. S.; Deng, L.; Hasegawa-Johnson, M.; He, X. D.; Random Features for Kernel Deep Convex Network, In 2013 IEEE International Conference on Acoustics, Speech and Signal Processing. IEEE, New York, 2013, 3143-3147.
- King, S.; Hasegawa-Johnson, M., Accurate Speech Segmentation by Mimicking Human Auditory Processing, In 2013 IEEE International Conference on Acoustics, Speech and Signal Processing. IEEE, New York, 2013, 8096-8100.
- Lin, K. H.; Zhuang, X. D.; Goudeseune, C.; King, S.; Hasegawa-Johnson, M.; Huang, T. S., Saliency-Maximized Audio Visualization and Efficient Audio-Visual Browsing for Faster-Than-Real-Time Human Acoustic Event Detection. ACM Transactions on Applied Perception 2013, 10, (4), DOI: 10.1145/2536764.2536773.
- Mertens, R.; Huang, P.-S.; Gottlieb, L.; Friedland, G.; Divakaran, A.; Hasegawa-Johnson, M., On the Application of Speaker Diarization to Audio Indexing of Non-Speech and Mixed Non-Speech/Speech Video Soundtracks. International Journal of Multimedia Data Engineering and Management 2013, 3, (3), 1-19.
- Sharma, H. V.; Hasegawa-Johnson, M., Acoustic Model Adaptation Using in-Domain Background Models for Dysarthric Speech Recognition. Computer Speech and Language 2013, 27, (6), 1147-1162, DOI: 10.1016/j.csl.2012.10.002.
2012
- Mahrt, T.; Cole, J.; Fleck, M.; Hasegawa-Johnson, M. F0 and the Perception of Prominence, Proceedings of Interspeech 2012, Portland, Oregon, 2012.
- Mahrt, T.; Cole, J.; Fleck, M.; Hasegawa-Johnson, M. Modeling Speaker Variation in Cues to Prominence Using the Bayesian Information Criterion, Proceedings of Speech Prosody 2012, Shanghai, 2012.
- Mathur, S.; Poole, M. S.; Feniosky, P. M.; Hasegawa-Johnson, M.; Contractor, N., Detecting Interaction Links in a Collaborating Group Using Manually Annotated Data. Social Networks 2012, DOI: doi:10.1016/j.socnet.2012.04.002.
- Mathur, S.; Poole, M. S.; Pena-Mora, F.; Hasegawa-Johnson, M.; Contractor, N., Detecting Interaction Links in a Collaborating Group Using Manually Annotated Data. Social Networks 2012, 34, (4), 515-526.
- Nam, H.; Mitra, V.; Tiede, M.; Hasegawa-Johnson, M.; Espy-Wilson, C.; Saltzman, E.; Goldstein, L., A Procedure for Estimating Gestural Scores from Speech Acoustics. Journal of the Acoustical Society of America 2012, 132, (6), 3980-3989.
- Ozbek, I. Y.; Hasegawa-Johnson, M.; Demirekler, M., On Improving Dynamic State Space Approaches to Articulatory Inversion with Map-Based Parameter Estimation. IEEE Transactions on Audio Speech and Language Processing 2012, 20, (1), 67-81.
- Rong, P. Y.; Loucks, T.; Kim, H.; Hasegawa-Johnson, M., Relationship between Kinematics, F2 Slope and Speech Intelligibility in Dysarthria Due to Cerebral Palsy. Clinical Linguistics & Phonetics 2012, 26, (9), 806-822.
- Tang, H.; Chu, S. M.; Hasegawa-Johnson, M.; Huang, T. S., Partially Supervised Speaker Clustering. IEEE Transactions on Pattern Analysis and Machine Intelligence 2012, 34, (5), 959-971.
2011
- Kim, H.; Hasegawa-Johnson, M.; Perlman, A., Vowel Contrast and Speech Intelligibility in Dysarthria. Folia Phoniatrica Et Logopaedica 2011, 63, (4), 187-194.
- Lobdell, B. E.; Allen, J. B.; Hasegawa-Johnson, M. A., Intelligibility predictors and neural representation of speech. Speech Communication 2011, 53, (2), 185-194.
- Ozbek, I. Y.; Hasegawa-Johnson, M.; Demirekler, M., Estimation of Articulatory Trajectories Based on Gaussian Mixture Model (Gmm) with Audio-Visual Information Fusion and Dynamic Kalman Smoothing. IEEE Transactions on Audio Speech and Language Processing 2011, 19, (5), 1180-1195.
- Zhuang, X. D.; Zhou, X.; Hasegawa-Johnson, M. A.; Huang, T. S., Efficient Object Localization with Variation-Normalized Gaussianized Vectors, In Intelligent Video Event Analysis and Understanding; Zhang, J., Shao, L., Zhang, L., Jones, G. A., Eds. 2011; Vol. 332, 93-109.
2010
- Kim, H.; Martin, K.; Hasegawa-Johnson, M.; Perlman, A., Frequency of Consonant Articulation Errors in Dysarthric Speech. Clinical Linguistics & Phonetics 2010, 24, (10), 759-770.
- Tang, H.; Hasegawa-Johnson, M.; Huang, T. S., Non-frontal View Facial Expression Recognition Based on Ergodic Hidden Markov Model Supervectors, IEEE International Conference on Multimedia & Expo, Singapore, 2010.
- Tang, H.; Hasegawa-Johnson, M.; Huang, T., A Novel Vector Representation of Stochastic Signals Based on Adapted Ergodic HMMs. IEEE Signal Processing Letters 2010, 17, (8), 715-718.
- Zhuang, X. D.; Zhou, X.; Hasegawa-Johnson, M. A.; Huang, T. S., Real-World Acoustic Event Detection. Pattern Recognition Letters 2010, 31, (12), 1543-1551.
- Zu, Y. H.; Hasegawa-Johnson, M.; Perlman, A.; Yang, Z., A Mathematical Model of Swallowing. Dysphagia 2010, 25, (4), 397-398.
2009
- Huang, T. S.; Hasegawa-Johnson, M. A.; Chu, S. M.; Zeng, Z.; Tang, H., Sensitive Talking Heads. IEEE Signal Processing Magazine 2009, 26, (4), 67-72.
- Yoon, P.; Huensch, A.; Juul, E.; Perkins, S.; Sproat, R.; Hasegawa-Johnson, M., Construction of a rated speech corpus of L2 learners' speech. CALICO Journal 2009, 26, (3), 662-673.
2008
- Chang, S. E.; Erickson, K. I.; Ambrose, N. G.; Hasegawa-Johnson, M. A.; Ludlow, C. L., Brain anatomy differences in childhood stuttering. Neuroimage 2008, 39, (3), 1333-1344.
- Kim, L. H.; Hasegawa-Johnson, M.; Lim, J. S.; Sung, K. M., Acoustic model for robustness analysis of optimal multipoint room equalization. Journal of the Acoustical Society of America 2008, 123, (4), 2043-2053.
- Tang, H.; Fu, Y.; Tu, J. L.; Hasegawa-Johnson, M.; Huang, T. S., Humanoid Audio-Visual Avatar With Emotive Text-to-Speech Synthesis. IEEE Transactions on Multimedia 2008, 10, (6), 969-981.
- Yoon, T.; Cole, J.; Hasegawa-Johnson, M. Detecting non-modal phonation in telephone speech, In Proceedings of Speech Prosody 2008, Campinas, Brazil, 2008.
2007
- Chen, K.; Hasegawa-Johnson, M.; Cole, J., A Factored Language Model for Prosody-Dependent Speech Recognition. In Speech Synthesis and Recognition, Kordic, V., Ed. Advanced Robotic Systems: 2007.
- Cole, J.; Kim, H.; Choi, H.; Hasegawa-Johnson, M., Prosodic effects on acoustic cues to stop voicing and place of articulation: Evidence from Radio News speech. Journal of Phonetics 2007, 35, (2), 180-209.
- Yoon, T.; Cole, J.; Hasegawa-Johnson, M. On the edge. Acoustic cues to layered prosodic domains, In Proceedings of the International Conference on Phonetic Sciences, Saarbrucken, Germany, 2007.
2006
- Zhang, T.; Hasegawa-Johnson, M.; Levinson, S. E., Cognitive state classification in a spoken tutorial dialogue system. Speech Communication 2006, 48, (6), 616-632.
- Zhang, T.; Hasegawa-Johnson, M.; Levinson, S. E., Extraction of pragmatic and semantic salience from spontaneous spoken English. Speech Communication 2006, 48, (3-4), 437-462.
-
2024
- Speech Accessibility Project’s three newest partners are dedicated to people with cerebral palsy
- Mark Hasegawa-Johnson on Apple's new AI-powered accessibility features
- Speech Accessibility Project funder Apple announces new accessibility features, including Eye Tracking, Music Haptics, and Vocal Shortcuts
- Speech Accessibility Project now sharing recordings, data
- Things to Know Right Now: Accessibility Project ft. Mark Hasegawa-Johnson, Ph.D. and Clarion Mendes
- Professor Mark Hasegawa-Johnson speaks on improving AI voice assistants
- Speech Accessibility Project expands to include ALS and cerebral palsy
- Speech Accessibility Project begins recruiting people who have had a stroke
- Voice recognition project recruiting adults with cerebral palsy
2023
- Grainger Engineers Explain: Speech Accessibility Project
- Speech Accessibility Project on the Parkinson's Experience Podcast
- UI researchers working to make speech-recognition technology more accessible
- U of I researchers working to make voice recognition more accessible, inclusive
- Beckman researchers lead initiative to make voice recognition technology more inclusive
- Speech Accessibility Project now recruiting adults with Down syndrome
- The Speech Accessibility Project could open doors, literally
- Poetry, phonemics, and accessible tech: Q&A with Mark Hasegawa-Johnson
- Beckman announces 2023 class of postdoctoral fellows
- Beckman Director's Seminar: Hasegawa-Johnson
- Hasegawa-Johnson discusses Speech Accessibility Project on This Week in Voice
- New AI Institute to Focus on the Speech Language Pathology Needs of Children
2022
- Amazon, Apple, Microsoft, Meta and Google to improve speech recognition for people with disabilities
- Top 5 tech companies in the world partner with U of I to improve voice recognition software
- Tech titans join forces with the University Of Illinois to launch new Speech Accessibility Project
- University of Illinois joins five technology industry leaders in Speech Accessibility Project
- Wearable tech offers up-close look at infant development
- Seven students win 2022 Beckman Institute Graduate Fellowships
2017
2014