Directory
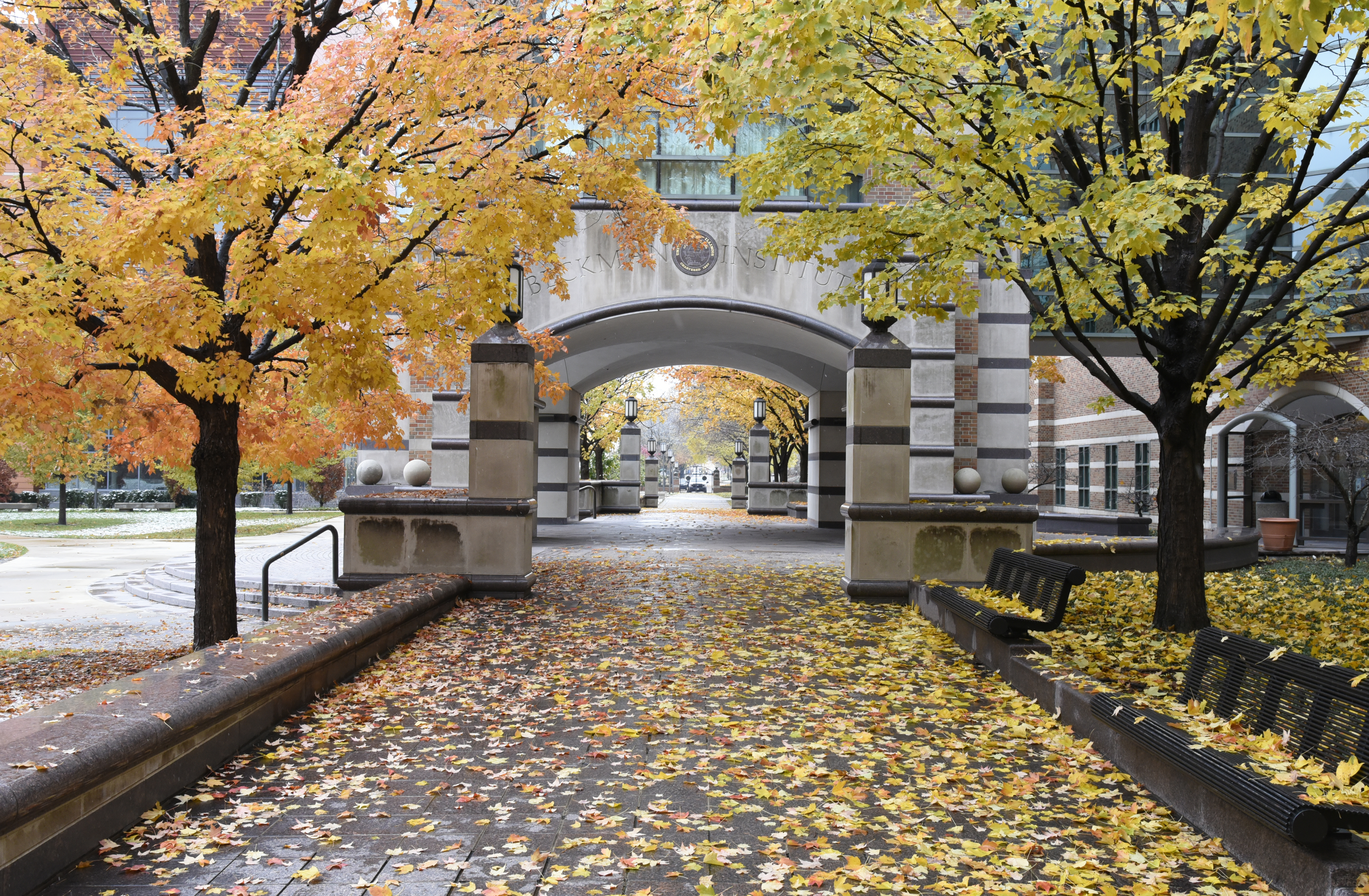
Want to swap out your latest headshot or add a new award? The Communications Office maintains the faculty biography, honors, and publications information included in Beckman Institute's online directory listings. You can request an update at any time.
Want to swap out your latest headshot or add a new award? The Communications Office maintains the faculty biography, honors, and publications information included in Beckman Institute's online directory listings. You can request an update at any time.
Beckman Institute for Advanced Science and Technology