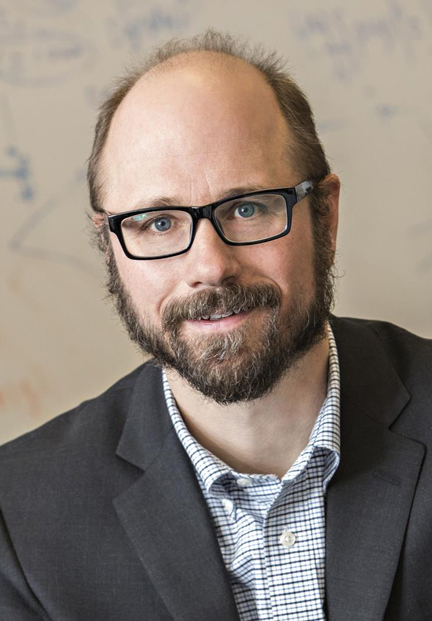
Status Part-time Faculty
Home Department of Bioengineering
Phone 333-1867
Email maa@illinois.edu
Address
-
Biography
Mark A. Anastasio is the Donald Biggar Willett Professor, and is also head of the Department of Bioengineering.
Education
- Ph.D., medical physics, The University of Chicago, 2001
-
Honors
- 2017: Elected as SPIE Fellow
- 2015: Elected as AIMBE Fellow
-
Research
Research areas:
Bioimaging at Multi-Scale
Research Interests:
BioPhotonics
Image reconstruction
Signal detection
Signal processing
Prof. Mark Anastasio is interested in researching computational and theoretical image science and pursuing the advancement of emerging imaging methods. Much of his research is aimed at the development of new imaging technologies, including photoacoustic tomography (PACT), ultrasound imaging tomography (USCT), and x-ray contrast imaging. Prof. Anastasio also conducts research related to the use of machine learning in imaging science. Topics include image reconstruction using machine learning and machine learning-based numerical observers, as well as computational methods for neuroimaging and the use of deep learning for image analysis.
-
2024
- A learning-based image reconstruction method for skull-induced aberrations compensation in transcranial photoacoustic computed tomography Huang, H. K., Kuo, J., Park, S., Villa, U., Wang, L. V. and Anastasio, M. A., 2024, Photons Plus Ultrasound: Imaging and Sensing 2024. Oraevsky, A. A. and Wang, L. V. (eds.). SPIE, 128420C. (Progress in Biomedical Optics and Imaging - Proceedings of SPIE; vol. 12842).
- A learning-based method for compensating 3D-2D model mismatch in ring-array ultrasound computed tomography Li, F., Villa, U. and Anastasio, M. A., 2024, Medical Imaging 2024: Ultrasonic Imaging and Tomography. Boehm, C. & Bottenus, N. (eds.). SPIE, 129321E. (Progress in Biomedical Optics and Imaging - Proceedings of SPIE; vol. 12932).
- A Test Statistic Estimation-Based Approach for Establishing Self-Interpretable CNN-Based Binary Classifiers Sengupta, S. and Anastasio, M. A., May 1 2024, In: IEEE transactions on medical imaging. 43, 5, p. 1753-1765 13 p.
- Application of learned ideal observers for estimating task-based performance bounds for computed imaging systems Li, K., Villa, U., Li, H. and Anastasio, M. A., Mar 1 2024, In: Journal of Medical Imaging. 11, 2, 026002.
- Assessing the capacity of a denoising diffusion probabilistic model to reproduce spatial context Deshpande, R., Ozbey, M., Li, H., Anastasio, M. A. and Brooks, F. J., 2024, (Accepted/In press) In: IEEE transactions on medical imaging. p. 1 1 p.
- Bayesian Cramér-Rao Bound Optimization of the Illumination Pattern in Quantitative Photoacoustic Computed Tomography Scope Crafts, E. D., Anastasio, M. A. and Villa, U., 2024, Medical Imaging 2024: Physics of Medical Imaging. Fahrig, R., Sabol, J. M. and Li, K. (eds.). SPIE, 129254A. (Progress in Biomedical Optics and Imaging - Proceedings of SPIE; vol. 12925).
- Context-aware deep learning enables high-efficacy localization of high concentration microbubbles for super-resolution ultrasound localization microscopy Shin, Y. R., Lowerison, M. R., Wang, Y., Chen, X., You, Q., Dong, Z., Anastasio, M. A. and Song, P., Dec 2024, In: Nature communications. 15, 1, 2932.
- Deep-learning-based image super-resolution of an end-expandable optical fiber probe for application in esophageal cancer diagnostics Zhang, X., Tan, M., Nabil, M., Shukla, R., Vasavada, S., Anandasabapathy, S., Anastasio, M. A. and Petrova, E., Apr 1 2024, In: Journal of biomedical optics. 29, 4, 046001.
- EVATOM: an optical, label-free, machine learning assisted embryo health assessment tool Goswami, N., Winston, N., Choi, W., Lai, N. Z. E., Arcanjo, R. B., Chen, X., Sobh, N., Nowak, R. A., Anastasio, M. A. and Popescu, G., Dec 2024, In: Communications biology. 7, 1, 268.
- Grand Challenges at the Interface of Engineering and Medicine Subramaniam, S., Akay, M., Anastasio, M. A., Bailey, V., Boas, D., Bonato, P., Chilkoti, A., Cochran, J. R., Colvin, V., Desai, T. A., Duncan, J. S., Epstein, F. H., Fraley, S., Giachelli, C., Grande-Allen, K. J., Green, J., Guo, X. E., Hilton, I. B., Humphrey, J. D. and Johnson, C. R. and 30 others, , 2024, In: IEEE Open Journal of Engineering in Medicine and Biology. 5, p. 1-13 13 p.
- High-Dimensional MR Reconstruction Integrating Subspace and Adaptive Generative Models Zhao, R., Peng, X., Kelkar, V. A., Anastasio, M. A. and Lam, F., Jun 1 2024, In: IEEE Transactions on Biomedical Engineering. 71, 6, p. 1969-1979 11 p.
- High-throughput photoacoustic tomography by integrated robotics and automation Marshall, N., Brecht, H. P., Thompson, W., Lawrence, D. J., Marshall, V., Toler, S., Emelianov, S., Yu, A., Anastasio, M., Villa, U., Maxwell, J. and Ermilov, S., 2024, Photons Plus Ultrasound: Imaging and Sensing 2024. Oraevsky, A. A. and Wang, L. V. (eds.). SPIE, 128420I. (Progress in Biomedical Optics and Imaging - Proceedings of SPIE; vol. 12842).
- Introducing the Special Issue Honoring Lihong V. Wang, Pioneer in Biomedical Optics Wang, X., Anastasio, M., Zhang, H., Sakadzic, S., Hu, S. and Gao, L., Jan 15 2024, In: Journal of biomedical optics. 29
- Investigation of a Learned Image Reconstruction Method for Three-Dimensional Quantitative Photoacoustic Tomography of the Breast Cam, R. M., Park, S., Villa, U. and Anastasio, M. A., 2024, Photons Plus Ultrasound: Imaging and Sensing 2024. Oraevsky, A. A. and Wang, L. V. (eds.). SPIE, 128420M. (Progress in Biomedical Optics and Imaging - Proceedings of SPIE; vol. 12842).
- Learned Full Waveform Inversion Incorporating Task Information for Ultrasound Computed Tomography Lozenski, L., Wang, H., Li, F., Anastasio, M., Wohlberg, B., Lin, Y. and Villa, U., 2024, In: IEEE Transactions on Computational Imaging. 10, p. 69-82 14 p.
- Learning a Semi-Analytic Reconstruction Method for Photoacoustic Computed Tomography with Hemispherical Measurement Geometries Chen, P., Park, S., Cam, R. M., Huang, H. K., Villa, U. and Anastasio, M. A., 2024, Photons Plus Ultrasound: Imaging and Sensing 2024. Oraevsky, A. A. & Wang, L. V. (eds.). SPIE, 128420U. (Progress in Biomedical Optics and Imaging - Proceedings of SPIE; vol. 12842).
- Learning a stable approximation of an existing but unknown inverse mapping: application to the half-time circular Radon transform Cam, R. M., Villa, U. and Anastasio, M. A., Aug 2024, In: Inverse Problems. 40, 8, 085002.
- Quantitative phase imaging techniques for measuring scattering properties of cells and tissues: a review-part II Goswami, N., Anastasio, M. A. and Popescu, G., Jun 1 2024, In: Journal of biomedical optics. 29, p. S22714
- Spatiotemporal image reconstruction to enable high-frame-rate dynamic photoacoustic tomography with rotating-gantry volumetric imagers Cam, R. M., Wang, C., Thompson, W., Ermilov, S. A., Anastasio, M. A. and Villa, U., Jan 15 2024, In: Journal of biomedical optics. 29, S11516.
- Transformer-based classifier with feature aggregation for cancer subtype classification on histopathological images Zhang, C., Fan, Z., Wang, Z., Sun, L., Hao, Y., Zhang, Z., Thorstad, W., Gay, H., Wang, X., Anastasio, M. A. and Li, H., 2024, Medical Imaging 2024: Image Processing. Colliot, O. & Mitra, J. (eds.). SPIE, 1292633. (Progress in Biomedical Optics and Imaging - Proceedings of SPIE; vol. 12926).
2023
- 3D full-waveform inversion in ultrasound computed tomography employing a ring-array Li, F., Villa, U., Duric, N. and Anastasio, M. A., 2023, Medical Imaging 2023: Ultrasonic Imaging and Tomography. Boehm, C. and Bottenus, N. (eds.). SPIE, 124700K. (Progress in Biomedical Optics and Imaging - Proceedings of SPIE; vol. 12470).
- A deep learning-based image reconstruction method for USCT that employs multimodality inputs Jeong, G., Li, F., Villa, U. and Anastasio, M. A., 2023, Medical Imaging 2023: Ultrasonic Imaging and Tomography. Boehm, C. and Bottenus, N. (eds.). SPIE, 124700M. (Progress in Biomedical Optics and Imaging - Proceedings of SPIE; vol. 12470).
- A Forward Model Incorporating Elevation-Focused Transducer Properties for 3-D Full-Waveform Inversion in Ultrasound Computed Tomography Li, F., Villa, U., Duric, N. and Anastasio, M. A., Oct 1 2023, In: IEEE Transactions on Ultrasonics, Ferroelectrics, and Frequency Control. 70, 10, p. 1339-1354 16 p.
- A machine learning approach to elucidating PFOS-induced alterations of repressive epigenetic marks in kidney cancer cells with single-cell imaging Liu, W., Zhang, X., Wen, Y., Anastasio, M. A. and Irudayaraj, J., Apr 2023, In: Environmental Advances. 11, 100344.
- Approximating the Hotelling observer with autoencoder-learned efficient channels for binary signal detection tasks Granstedt, J. L., Zhou, W. and Anastasio, M. A., Sep 1 2023, In: Journal of Medical Imaging. 10, 5, 055501.
- Artificial confocal microscopy for deep label-free imaging Chen, X., Kandel, M. E., He, S., Hu, C., Lee, Y. J., Sullivan, K., Tracy, G., Chung, H. J., Kong, H. J., Anastasio, M. and Popescu, G., Mar 2023, In: Nature Photonics. 17, 3, p. 250-258 9 p.
- Assessing the Ability of Generative Adversarial Networks to Learn Canonical Medical Image Statistics Kelkar, V. A., Gotsis, D. S., Brooks, F. J., Prabhat, K. C., Myers, K. J., Zeng, R. and Anastasio, M. A., Jun 1 2023, In: IEEE transactions on medical imaging. 42, 6, p. 1799-1808 10 p.
- Attention-Based CNN-BiLSTM for Sleep State Classification of Spatiotemporal Wide-Field Calcium Imaging Data Zhang, X., Landsness, E. C., Culver, J. P., Lee, J. M. and Anastasio, M. A., 2023, Neural Imaging and Sensing 2023. Luo, Q., Ding, J. & Fu, L. (eds.). SPIE, 123650B. (Progress in Biomedical Optics and Imaging - Proceedings of SPIE; vol. 12365).
- Contrast-Free Super-Resolution Power Doppler (CS-PD) Based on Deep Neural Networks You, Q., Lowerison, M. R., Shin, Y., Chen, X., Sekaran, N. V. C., Dong, Z., Llano, D. A., Anastasio, M. A. and Song, P., Oct 1 2023, In: IEEE Transactions on Ultrasonics, Ferroelectrics, and Frequency Control. 70, 10, p. 1355-1368 14 p.
- Dynamic Image Reconstruction to Monitor Tumor Vascular Perfusion in Small Animals using 3D Photoacoustic Computed-Tomography Imagers with Rotating Gantries Cam, R. M., Wang, C., Park, S., Thompson, W., Ermilov, S. A., Anastasio, M. A. and Villa, U., 2023, Photons Plus Ultrasound: Imaging and Sensing 2023. Oraevsky, A. A. and Wang, L. V. (eds.). SPIE, 123790F. (Progress in Biomedical Optics and Imaging - Proceedings of SPIE; vol. 12379).
- Estimating task-based performance bounds for image reconstruction methods by use of learned-ideal observers Li, K., Zhou, W., Li, H. and Anastasio, M. A., 2023, Medical Imaging 2023: Image Perception, Observer Performance, and Technology Assessment. Mello-Thoms, C. R. and Chen, Y. (eds.). SPIE, 124670I. (Progress in Biomedical Optics and Imaging - Proceedings of SPIE; vol. 12467).
- Evaluating generative stochastic image models using task-based image quality measures Kelkar, V. A., Gotsis, D. S., Deshpande, R., Brooks, F. J., Prabhat, K. C., Myers, K. J., Zeng, R. and Anastasio, M. A., 2023, Medical Imaging 2023: Image Perception, Observer Performance, and Technology Assessment. Mello-Thoms, C. R. and Chen, Y. (eds.). SPIE, 1246717. (Progress in Biomedical Optics and Imaging - Proceedings of SPIE; vol. 12467).
- Evaluation of U-Net for Time-Domain Full Waveform Inversion Improvement Anwar, S., Yunker, A., Kettimuthu, R., Anastasio, M. A., Villa, U. and He, J., 2023, Structural Health Monitoring 2023: Designing SHM for Sustainability, Maintainability, and Reliability - Proceedings of the 14th International Workshop on Structural Health Monitoring. Farhangdoust, S., Guemes, A. and Chang, F.-K. (eds.). DEStech Publications, p. 2197-2204 8 p. (Structural Health Monitoring 2023: Designing SHM for Sustainability, Maintainability, and Reliability - Proceedings of the 14th International Workshop on Structural Health Monitoring).
- Ideal Observer Computation by Use of Markov-Chain Monte Carlo with Generative Adversarial Networks Zhou, W., Villa, U. and Anastasio, M. A., Dec 1 2023, In: IEEE transactions on medical imaging. 42, 12, p. 3715-3724 10 p.
- Investigating the robustness of a deep learning-based method for quantitative phase retrieval from propagation-based x-ray phase contrast measurements under laboratory conditions Deshpande, R., Avachat, A., Brooks, F. J. and Anastasio, M. A., Apr 21 2023, In: Physics in medicine and biology. 68, 8, 085005.
- Memory-efficient self-supervised learning of null space projection operators Zhai, A. J., Kuo, J., Anastasio, M. A. and Villa, U., 2023, Medical Imaging 2023: Physics of Medical Imaging. Yu, L., Fahrig, R. and Sabol, J. M. (eds.). SPIE, 124631I. (Progress in Biomedical Optics and Imaging - Proceedings of SPIE; vol. 12463).
- Photoacoustics special issue ‘Photoacoustic image reconstruction: Theory and practice’ Cox, B. and Anastasio, M., Apr 2023, In: Photoacoustics. 30, 100461.
- Semi-supervised Contrastive Learning for White Blood Cell Segmentation from Label-free Quantitative Phase Imaging Sengupta, S., Fanous, M., Li, H. and Anastasio, M. A., 2023, Medical Imaging 2023: Digital and Computational Pathology. Tomaszewski, J. E. and Ward, A. D. (eds.). SPIE, 124710E. (Progress in Biomedical Optics and Imaging - Proceedings of SPIE; vol. 12471).
- Stochastic three-dimensional numerical phantoms to enable computational studies in quantitative optoacoustic computed tomography of breast cancer Park, S., Villa, U., Li, F., Cam, R. M., Oraevsky, A. A. and Anastasio, M. A., Jun 1 2023, In: Journal of biomedical optics. 28, 6, 066002.
- The stochastic digital human is now enrolling for in silico imaging trials—methods and tools for generating digital cohorts Badano, A., Lago, M. A., Sizikova, E., Delfino, J. G., Guan, S., Anastasio, M. A. and Sahiner, B., Oct 1 2023, In: Progress in Biomedical Engineering. 5, 4, 042002.
2022
- 3-D Stochastic Numerical Breast Phantoms for Enabling Virtual Imaging Trials of Ultrasound Computed Tomography Li, F., Villa, U., Park, S. and Anastasio, M. A., Jan 1 2022, In: IEEE Transactions on Ultrasonics, Ferroelectrics, and Frequency Control. 69, 1, p. 135-146 12 p.
- A Hybrid Approach for Approximating the Ideal Observer for Joint Signal Detection and Estimation Tasks by Use of Supervised Learning and Markov-Chain Monte Carlo Methods Li, K., Zhou, W., Li, H. and Anastasio, M. A., May 1 2022, In: IEEE transactions on medical imaging. 41, 5, p. 1114-1124 11 p.
- A learned filtered backprojection method for use with half-time circular radon transform data Cam, R. M., Villa, U. & Anastasio, M. A., 2022, Medical Imaging 2022: Physics of Medical Imaging. Zhao, W. and Yu, L. (eds.). SPIE, 1203134. (Progress in Biomedical Optics and Imaging - Proceedings of SPIE; vol. 12031).
- A Memory-Efficient Self-Supervised Dynamic Image Reconstruction Method Using Neural Fields Lozenski, L., Anastasio, M. A. and Villa, U., 2022, In: IEEE Transactions on Computational Imaging. 8, p. 879-892 14 p.
- A Multivariate Functional Connectivity Approach to Mapping Brain Networks and Imputing Neural Activity in Mice Brier, L. M., Zhang, X., Bice, A. R., Gaines, S. H., Landsness, E. C., Lee, J. M., Anastasio, M. A. and Culver, J. P., Apr 15 2022, In: Cerebral Cortex. 32, 8, p. 1593-1607 15 p.
- A Task-informed Model Training Method for Deep Neural Network-based Image Denoising Li, K., Li, H. and Anastasio, M. A., 2022, Medical Imaging 2022: Image Perception, Observer Performance, and Technology Assessment. Mello-Thoms, C. R., Mello-Thoms, C. R. and Taylor-Phillips, S. (eds.). SPIE, 1203510. (Progress in Biomedical Optics and Imaging - Proceedings of SPIE; vol. 12035).
- Analyzing Neural Networks Applied to an Anatomical Simulation of the Breast Abbey, C. K., Sengupta, S., Zhou, W., Badal, A., Zeng, R., Samuelson, F. W., Eckstein, M. P., Myers, K. J., Anastasio, M. A. & Brankov, J. G., 2022, Medical Imaging 2022: Image Perception, Observer Performance, and Technology Assessment. Mello-Thoms, C. R., Mello-Thoms, C. R. and Taylor-Phillips, S. (eds.). SPIE, 1203505. (Progress in Biomedical Optics and Imaging - Proceedings of SPIE; vol. 12035). Research output: Chapter in Book/Report/Conference proceeding › Conference contribution
- Application of DatasetGAN in medical imaging: preliminary studies Fan, Z., Kelkar, V., Anastasio, M. A. and Li, H., 2022, Medical Imaging 2022: Image Processing. Colliot, O., Isgum, I., Landman, B. A. and Loew, M. H. (eds.). SPIE, 120321O. (Progress in Biomedical Optics and Imaging - Proceedings of SPIE; vol. 12032).
- Automated sleep state classification of wide-field calcium imaging data via multiplex visibility graphs and deep learning Zhang, X., Landsness, E. C., Chen, W., Miao, H., Tang, M., Brier, L. M., Culver, J. P., Lee, J. M. and Anastasio, M. A., Jan 15 2022, In: Journal of Neuroscience Methods. 366, 109421.
- Cell Cycle Stage Classification Using Phase Imaging with Computational Specificity He, Y. R., He, S., Kandel, M. E., Lee, Y. J., Hu, C., Sobh, N., Anastasio, M. A. and Popescu, G., Apr 20 2022, In: ACS Photonics. 9, 4, p. 1264-1273 10 p.
- Computing a projection operator onto the null space of a linear imaging operator: tutorial Kuo, J., Granstedt, J., Villa, U. and Anastasio, M. A., Mar 1 2022, In: Journal of the Optical Society of America A: Optics and Image Science, and Vision. 39, 3, p. 470-481 12 p.
- Dynamic Heterochromatin States in Anisotropic Nuclei of Cells on Aligned Nanofibers Liu, W., Padhi, A., Zhang, X., Narendran, J., Anastasio, M. A., Nain, A. S. and Irudayaraj, J., Jul 26 2022, In: ACS Nano. 16, 7, p. 10754-10767 14 p.
- Evaluating Procedures for Establishing Generative Adversarial Network-based Stochastic Image Models in Medical Imaging Kelkar, V. A., Gotsis, D. S., Brooks, F. J., Myers, K. J., Prabhat, K. C., Zeng, R. and Anastasio, M. A., 2022, Medical Imaging 2022: Image Perception, Observer Performance, and Technology Assessment. Mello-Thoms, C. R., Mello-Thoms, C. R. and Taylor-Phillips, S. (eds.). SPIE, 120350O. (Progress in Biomedical Optics and Imaging - Proceedings of SPIE; vol. 12035).
- Evaluating the Capacity of Deep Generative Models to Reproduce Measurable High-order Spatial Arrangements in Diagnostic Images Deshpande, R., Anastasio, M. A. and Brooks, F. J., 2022, Medical Imaging 2022: Image Processing. Colliot, O., Isgum, I., Landman, B. A. and Loew, M. H. (eds.). SPIE, 120321X. (Progress in Biomedical Optics and Imaging - Proceedings of SPIE; vol. 12032).
- Image Reconstruction in Phase-Contrast CT with Shortened Scans Zhang, Z., Chen, B., Xia, D., Sidky, E. Y., Anastasio, M. and Pan, X., 2022, 7th International Conference on Image Formation in X-Ray Computed Tomography. Stayman, J. W. (ed.). SPIE, 123042A. (Proceedings of SPIE - The International Society for Optical Engineering; vol. 12304).
- Investigation of Adversarial Robust Training for Establishing Interpretable CNN-based Numerical Observers Sengupta, S., Abbey, C. K., Li, K. & Anastasio, M. A., 2022, Medical Imaging 2022: Image Perception, Observer Performance, and Technology Assessment. Mello-Thoms, C. R., Mello-Thoms, C. R. and Taylor-Phillips, S. (eds.). SPIE, 1203514. (Progress in Biomedical Optics and Imaging - Proceedings of SPIE; vol. 12035).
- Investigation of an elevation-focused transducer model to enable accurate three-dimensional full-waveform inversion in ultrasound computed tomography Li, F., Villa, U., Duric, N. and Anastasio, M. A., 2022, Medical Imaging 2022: Ultrasonic Imaging and Tomography. Bottenus, N. and Ruiter, N. V. (eds.). SPIE, 120380S. (Progress in Biomedical Optics and Imaging - Proceedings of SPIE; vol. 12038).
- Investigation of an elevation-focused transducer model to enable accurate three-dimensional full-waveform inversion in ultrasound computed tomography Li, F., Villa, U., Duric, N. and Anastasio, M. A., 2022, Medical Imaging 2022: Ultrasonic Imaging and Tomography. Bottenus, N. and Ruiter, N. V. (eds.). SPIE, 120380S. (Progress in Biomedical Optics and Imaging - Proceedings of SPIE; vol. 12038).
- Learning stochastic object models from medical imaging measurements by use of advanced ambient generative adversarial networks Zhou, W., Bhadra, S., Brooks, F. J., Li, H. and Anastasio, M. A., Jan 1 2022, In: Journal of Medical Imaging. 9, 1, 015503.
- Live-dead assay on unlabeled cells using phase imaging with computational specificity Hu, C., He, S., Lee, Y. J., He, Y., Kong, E. M., Li, H., Anastasio, M. A. and Popescu, G., Dec 2022, In: Nature communications. 13, 1, 713.
- Neural fields for dynamic imaging Lozenski, L., Anastasio, M. and Villa, U., 2022, Medical Imaging 2022: Physics of Medical Imaging. Zhao, W. and Yu, L. (eds.). SPIE, 120310X. (Progress in Biomedical Optics and Imaging - Proceedings of SPIE; vol. 12031).
- Normalization of optical fluence distribution for three-dimensional functional optoacoustic tomography of the breast Park, S., Brooks, F. J., Villa, U., Su, R., Anastasio, M. A. and Oraevsky, A. A., Mar 1 2022, In: Journal of biomedical optics. 27, 3, p. 36001 1 p.
- Preliminary Study of Image Reconstruction from Sparse-View Data in Phase-Contrast CT Zhang, Z., Chen, B., Xia, D., Sikdy, E. Y., Anastasio, M. and Pan, X., 2022, 2022 IEEE NSS/MIC RTSD - IEEE Nuclear Science Symposium, Medical Imaging Conference and Room Temperature Semiconductor Detector Conference. Institute of Electrical and Electronics Engineers Inc., (2022 IEEE NSS/MIC RTSD - IEEE Nuclear Science Symposium, Medical Imaging Conference and Room Temperature Semiconductor Detector Conference).
- Prior image-based medical image reconstruction using a style-based generative adversarial network Kelkar, V. A. and Anastasio, M. A., 2022, Medical Imaging 2022: Physics of Medical Imaging. Zhao, W. and Yu, L. (eds.). SPIE, 120310Z. (Progress in Biomedical Optics and Imaging - Proceedings of SPIE; vol. 12031).
- White blood cell detection, classification and analysis using phase imaging with computational specificity (PICS) Fanous, M. J., He, S., Sengupta, S., Tangella, K., Sobh, N., Anastasio, M. A. and Popescu, G., Dec 2022, In: Scientific reports. 12, 1, 20043.
2021
- 3D microscopy and deep learning reveal the heterogeneity of crown-like structure microenvironments in intact adipose tissue Geng, J., Zhang, X., Prabhu, S., Shahoei, S. H., Nelson, E. R., Swanson, K. S., Anastasio, M. A. and Smith, A. M., Feb 17 2021, In: Science Advances. 7, 8, eabe2480.
- A hybrid channelized Hotelling observer for estimating the ideal linear observer for total-variation-based image reconstruction Phillips, J. P., Sidky, E. Y., Ongie, G., Zhou, W., Cruz-Bastida, J. P., Reiser, I. S., Anastasio, M. A. and Pan, X., 2021, Medical Imaging 2021: Image Perception, Observer Performance, and Technology Assessment. Samuelson, F. W. and Taylor-Phillips, S. (eds.). SPIE, 115990D. (Progress in Biomedical Optics and Imaging - Proceedings of SPIE; vol. 11599).
- A signal detection model for quantifying overregularization in nonlinear image reconstruction Sidky, E. Y., Phillips, J. P., Zhou, W., Ongie, G., Cruz-Bastida, J. P., Reiser, I. S., Anastasio, M. A. and Pan, X., Oct 2021, In: Medical Physics. 48, 10, p. 6312-6323 12 p.
- Advancing the AmbientGAN for learning stochastic object models Zhou, W., Bhadra, S., Brooks, F. J., Granstedt, J. L., Li, H. and Anastasio, M. A., 2021, Medical Imaging 2021: Image Perception, Observer Performance, and Technology Assessment. Samuelson, F. W. and Taylor-Phillips, S. (eds.). SPIE, 115990A. (Progress in Biomedical Optics and Imaging - Proceedings of SPIE; vol. 11599).
- An optical, endoscopic brush for high-yield diagnostics in esophageal cancer Anandasabapathy, S., Zhang, X., Anastasio, M., Richards-Kortum, R. and Petrova, E., 2021, Endoscopic Microscopy XVI. Tearney, G. J., Wang, T. D. and Suter, M. J. (eds.). SPIE, 116200B. (Progress in Biomedical Optics and Imaging - Proceedings of SPIE; vol. 11620).
- Assessing the Impact of Deep Neural Network-Based Image Denoising on Binary Signal Detection Tasks Li, K., Zhou, W., Li, H. and Anastasio, M. A., Sep 2021, In: IEEE transactions on medical imaging. 40, 9, p. 2295-2305 11 p., 9419965.
- Compressible Latent-Space Invertible Networks for Generative Model-Constrained Image Reconstruction Kelkar, V. A., Bhadra, S. and Anastasio, M. A., 2021, In: IEEE Transactions on Computational Imaging. 7, p. 209-223 15 p., 9318016.
- Decoding visual information from high-density diffuse optical tomography neuroimaging data Tripathy, K., Markow, Z. E., Fishell, A. K., Sherafati, A., Burns-Yocum, T. M., Schroeder, M. L., Svoboda, A. M., Eggebrecht, A. T., Anastasio, M. A., Schlaggar, B. L. and Culver, J. P., Feb 1 2021, In: NeuroImage. 226, 117516.
- Deeply-supervised density regression for automatic cell counting in microscopy images He, S., Minn, K. T., Solnica-Krezel, L., Anastasio, M. A. and Li, H., Feb 2021, In: Medical Image Analysis. 68, 101892.
- Impact of deep learning-based image super-resolution on binary signal detection Zhang, X., Kelkar, V. A., Granstedt, J., Li, H. and Anastasio, M. A., Nov 1 2021, In: Journal of Medical Imaging. 8, 6, 065501.
- Learning a projection operator onto the null space of a linear imaging operator Kuo, J., Granstedt, J., Villa, U. and Anastasio, M. A., 2021, Medical Imaging 2021: Physics of Medical Imaging. Bosmans, H., Zhao, W. and Yu, L. (eds.). SPIE, 115953X. (Progress in Biomedical Optics and Imaging - Proceedings of SPIE; vol. 11595).
- Medical image reconstruction using compressible latent space invertible networks Kelkar, V. A., Bhadra, S. and Anastasio, M. A., 2021, Medical Imaging 2021: Physics of Medical Imaging. Bosmans, H., Zhao, W. and Yu, L. (eds.). SPIE, 115951S. (Progress in Biomedical Optics and Imaging - Proceedings of SPIE; vol. 11595).
- Model-based optical and acoustical compensation for photoacoustic tomography of heterogeneous mediums Pattyn, A., Mumm, Z., Alijabbari, N., Duric, N., Anastasio, M. A. and Mehrmohammadi, M., Sep 2021, In: Photoacoustics. 23, 100275.
- On Hallucinations in Tomographic Image Reconstruction Bhadra, S., Kelkar, V. A., Brooks, F. J. and Anastasio, M. A., Nov 1 2021, In: IEEE transactions on medical imaging. 40, 11, p. 3249-3260 12 p.
- Prior Image-Constrained Reconstruction using Style-Based Generative Models Kelkar, V. A. and Anastasio, M. A., 2021, Proceedings of the 38th International Conference on Machine Learning, ICML 2021. ML Research Press, p. 5367-5377 11 p. (Proceedings of Machine Learning Research; vol. 139).
- SlabGAN: A method for generating efficient 3D anisotropic medical volumes using generative adversarial networks Granstedt, J. L., Kelkar, V. A., Zhou, W. and Anastasio, M. A., 2021, Medical Imaging 2021: Image Processing. Isgum, I. and Landman, B. A. (eds.). SPIE, 1159617. (Progress in Biomedical Optics and Imaging - Proceedings of SPIE; vol. 11596).
- Special Series Guest Editorial: Artificial Intelligence and Machine Learning in Biomedical Optics Shabestri, B., Anastasio, M. A., Fei, B. and Leblond, F., May 1 2021, In: Journal of biomedical optics. 26, 5, 052901.
- Supervised learning-based ideal observer approximation for joint detection and estimation tasks Li, K., Zhou, W., Li, H. and Anastasio, M. A., 2021, Medical Imaging 2021: Image Perception, Observer Performance, and Technology Assessment. Samuelson, F. W. and Taylor-Phillips, S. (eds.). SPIE, 115990F. (Progress in Biomedical Optics and Imaging - Proceedings of SPIE; vol. 11599).
- Task-based evaluation of deep image super-resolution in medical imaging Kelkar, V. A., Zhang, X., Granstedt, J., Li, H. and Anastasio, M. A., 2021, Medical Imaging 2021: Image Perception, Observer Performance, and Technology Assessment. Samuelson, F. W. and Taylor-Phillips, S. (eds.). SPIE, 115990X. (Progress in Biomedical Optics and Imaging - Proceedings of SPIE; vol. 11599).
- Task-based performance evaluation of deep neural network-based image denoising Li, K., Zhou, W., Li, H. and Anastasio, M. A., 2021, Medical Imaging 2021: Image Perception, Observer Performance, and Technology Assessment. Samuelson, F. W. and Taylor-Phillips, S. (eds.). SPIE, 115990L. (Progress in Biomedical Optics and Imaging - Proceedings of SPIE; vol. 11599).
2020
- A partial-dithering strategy for edge-illumination X-ray phase-contrast tomography enabled by a joint reconstruction method Chen, Y., Hagen, C. K., Olivo, A. and Anastasio, M. A., May 21 2020, In: Physics in medicine and biology. 65, 10, 105007.
- A preclinical small animal imaging platform combining multi-angle photoacoustic and fluorescence projections into co-registered 3D maps Thompson, W., Yu, A., Dumani, D. S., Cook, J., Anastasio, M. A., Emelianov, S. Y. and Ermilov, S. A., 2020, Photons Plus Ultrasound: Imaging and Sensing 2020. Oraevsky, A. A. and Wang, L. V. (eds.). SPIE, 112400L. (Progress in Biomedical Optics and Imaging - Proceedings of SPIE; vol. 11240).
- Approximating the Ideal Observer for Joint Signal Detection and Localization Tasks by use of Supervised Learning Methods Zhou, W., Li, H. and Anastasio, M. A., Dec 2020, In: IEEE transactions on medical imaging. 39, 12, p. 3992-4000 9 p., 9139307.
- Comparison of data-acquisition designs for single-shot edge-illumination X-ray phase-contrast tomography Chen, Y., Zhou, W., Hagen, C. K., Olivo, A. and Anastasio, M. A., 2020, In: Optics Express. 28, 1, p. 1-19 19 p.
- Gold nanorods enable noninvasive longitudinal monitoring of hydrogels in vivo with photoacoustic tomography Shrestha, B., Stojkova, K., Yi, R., Anastasio, M. A., Ye, J. Y. and Brey, E. M., Nov 2020, In: Acta Biomaterialia. 117, p. 374-383 10 p.
- High-resolution transcriptional and morphogenetic profiling of cells from micropatterned human esc gastruloid cultures Minn, K. T., Fu, Y. C., He, S., Dietmann, S., George, S. C., Anastasio, M. A., Morris, S. A. and Solnica-Krezel, L., Oct 2020, In: eLife. 9, p. 1-34 34 p., e59445.
- Improved breast cancer diagnosis using ultrasound guided diffuse optical tomography Shihab Uddin, K. M., Zhang, M., Brooks, F., Anastasio, M. and Zhu, Q., 2020, Clinical and Translational Biophotonics, Translational 2020. Optica Publishing Group (formerly OSA), (Optics InfoBase Conference Papers; vol. Part F178-Translational-2020).
- Iterative image reconstruction in transcranial photoacoustic tomography based on the elastic wave equation Poudel, J., Na, S., Wang, L. V. and Anastasio, M. A., 2020, In: Physics in medicine and biology. 65, 5, 055009.
- Joint reconstruction of initial pressure distribution and spatial distribution of acoustic properties of elastic media with application to transcranial photoacoustic tomography Poudel, J. and Anastasio, M. A., Dec 2020, In: Inverse Problems. 36, 12, 124007.
- Learning efficient channels with a dual loss autoencoder Granstedt, J. L., Zhou, W. and Anastasio, M. A., 2020, Medical Imaging 2020: Image Perception, Observer Performance, and Technology Assessment. Samuelson, F. W. and Taylor-Phillips, S. (eds.). SPIE, 113160C. (Progress in Biomedical Optics and Imaging - Proceedings of SPIE; vol. 11316).
- Learning numerical observers using unsupervised domain adaptation He, S., Zhou, W., Li, H. and Anastasio, M. A., 2020, Medical Imaging 2020: Image Perception, Observer Performance, and Technology Assessment. Samuelson, F. W. and Taylor-Phillips, S. (eds.). SPIE, 113160W. (Progress in Biomedical Optics and Imaging - Proceedings of SPIE; vol. 11316).
- Markov-Chain Monte Carlo approximation of the Ideal Observer using generative adversarial networks Zhou, W. and Anastasio, M. A., 2020, Medical Imaging 2020: Image Perception, Observer Performance, and Technology Assessment. Samuelson, F. W. and Taylor-Phillips, S. (eds.). SPIE, 113160D. (Progress in Biomedical Optics and Imaging - Proceedings of SPIE; vol. 11316).
- Medical image reconstruction with image-adaptive priors learned by use of generative adversarial networks Bhadra, S., Zhou, W. and Anastasio, M. A., 2020, Medical Imaging 2020: Physics of Medical Imaging. Chen, G.-H. and Bosmans, H. (eds.). SPIE, 113120V. (Progress in Biomedical Optics and Imaging - Proceedings of SPIE; vol. 11312).
- Optimal breast cancer diagnostic strategy using combined ultrasound and diffuse optical tomography Shihab Uddin, K. M., Zhang, M., Anastasio, M. and Zhu, Q., May 1 2020, In: Biomedical Optics Express. 11, 5, p. 2722-2737 16 p.
- Photoacoustic Imaging in Tissue Engineering and Regenerative Medicine Shrestha, B., Deluna, F., Anastasio, M. A., Yong Ye, J. and Brey, E. M., Feb 2020, In: Tissue Engineering - Part B: Reviews. 26, 1, p. 79-102 24 p.
- Progressively-Growing AmbientGANs for learning stochastic object models from imaging measurements Zhou, W., Bhadra, S., Brooks, F. J., Li, H. and Anastasio, M. A., 2020, Medical Imaging 2020: Image Perception, Observer Performance, and Technology Assessment. Samuelson, F. W. and Taylor-Phillips, S. (eds.). SPIE, 113160Q. (Progress in Biomedical Optics and Imaging - Proceedings of SPIE; vol. 11316).
- X-ray CT in Phase Contrast Enhancement Geometry of Alginate Microbeads in a Whole-Animal Model Brown, J., Somo, S., Brooks, F., Komarov, S., Zhou, W., Anastasio, M. and Brey, E., Mar 1 2020, In: Annals of Biomedical Engineering. 48, 3, p. 1016-1024 9 p.
2019
- A survey of computational frameworks for solving the acoustic inverse problem in three-dimensional photoacoustic computed tomography Poudel, J., Lou, Y. and Anastasio, M. A., Jul 18 2019, In: Physics in medicine and biology. 64, 14, 14TR01.
- Advancements in image reconstruction for photoacoustic computed tomography (Conference Presentation) Anastasio, M. A., Mar 4 2019, p. 29.
- All-reflective ring illumination system for photoacoustic tomography Alshahrani, S. S., Yan, Y., Alijabbari, N., Pattyn, A., Avrutsky, I., Malyarenko, E., Poudel, J., Anastasio, M. and Mehrmohammadi, M., Apr 1 2019, In: Journal of biomedical optics. 24, 4, 046004.
- Analysis of the Use of Unmatched Backward Operators in Iterative Image Reconstruction With Application to Three-Dimensional Optoacoustic Tomography Lou, Y., Park, S., Anis, F., Su, R., Oraevsky, A. A. and Anastasio, M. A., Sep 1 2019, In: IEEE Transactions on Computational Imaging. 5, 3, p. 437-449 13 p., 8625498.
- Approximating the Ideal Observer and Hotelling Observer for Binary Signal Detection Tasks by Use of Supervised Learning Methods Zhou, W., Li, H. and Anastasio, M. A., Oct 2019, In: IEEE transactions on medical imaging. 38, 10, p. 2456-2468 13 p., 8691467.
- Autoencoder embedding of task-specific information Granstedt, J., Zhou, W. and Anastasio, M. A., Mar 4 2019, p. 6.
- Automatic microscopic cell counting by use of deeply-supervised density regression model He, S., Minn, K. T., Solnica-krezel, L., Anastasio, M. and Li, H., Mar 18 2019, p. 19.
- Automatic microscopic cell counting by use of unsupervised adversarial domain adaptation and supervised density regression He, S., Minn, K. T., Solnica-krezel, L., Li, H. and Anastasio, M., Mar 18 2019, p. 2.
- Compensation for non-uniform illumination and optical fluence attenuation in three-dimensional optoacoustic tomography of the breast Park, S., Oraevsky, A. A., Su, R. and Anastasio, M. A., Mar 8 2019, p. 180.
- Compensation of 3D-2D model mismatch in ultrasound computed tomography with the aid of convolutional neural networks (Conference Presentation) Poudel, J., Forte, L. A. and Anastasio, M. A., Mar 15 2019, p. 6.
- Joint-reconstruction-enabled partial-dithering strategy for edge-illumination x-ray phase-contrast tomography Chen, Y. and Anastasio, M. A., Mar 1 2019, p. 60.
- Learning stochastic object model from noisy imaging measurements using AmbientGANs Zhou, W., Bhadra, S., Brooks, F. and Anastasio, M. A., Mar 4 2019, p. 21.
- Learning the Hotelling observer for SKE detection tasks by use of supervised learning methods Zhou, W., Li, H. and Anastasio, M. A., Mar 4 2019, p. 7.
- Learning the ideal observer for joint detection and localization tasks by use of convolutional neural networks Zhou, W. and Anastasio, M. A., Mar 4 2019, p. 8.
- Preclinical small animal imaging platform providing co-registered 3D maps of photoacoustic response and fluorescence Dumani, D. S., Yu, A., Thompson, W., Brecht, H.-P., Ivanov, V., Anastasio, M. A., Cook, J. R., Ermilov, S. A. and Emelianov, S. Y., Mar 19 2019, p. 181.
- Quantification of image texture in X-ray phase-contrast-enhanced projection images of in vivo mouse lungs observed at varied inflation pressures Brooks, F. J., Gunsten, S. P., Vasireddi, S. K., Brody, S. L. and Anastasio, M. A., 2019, In: Physiological Reports. 7, 16, e14208.
- Reconstruction-Aware Imaging System Ranking by Use of a Sparsity-Driven Numerical Observer Enabled by Variational Bayesian Inference Chen, Y., Lou, Y., Wang, K., Kupinski, M. A. and Anastasio, M. A., May 2019, In: IEEE transactions on medical imaging. 38, 5, p. 1251-1262 12 p., 8542729.
- Special Section Guest Editorial: Celebrating the Exponential Growth of Optoacoustic/Photoacoustic Imaging Beard, P., Anastasio, M., Oraevsky, A. A. and Wang, L. V., Dec 1 2019, In: Journal of biomedical optics. 24, 12, 121901.
- Task-based image quality assessment in radiation therapy: Initial characterization and demonstration with computer-simulation study Dolly, S. R., Lou, Y., Anastasio, M. A. and Li, H., Jul 18 2019, In: Physics in medicine and biology. 64, 14, 145020.
- Treatment Outcome Prediction for Cancer Patients based on Radiomics and Belief Function Theory Wu, J., Lian, C., Ruan, S., Mazur, T. R., Mutic, S., Anastasio, M. A., Grigsby, P. W., Vera, P. and Li, H., Mar 2019, In: IEEE Transactions on Radiation and Plasma Medical Sciences. 3, 2, p. 216-224 9 p., 8474291.
- X-ray phase contrast imaging of soft biomaterials in a whole-animal model Brown, J., Somo, S., Brooks, F., Komarov, S., Zhou, W., Anastasio, M. and Brey, E., 2019, Society for Biomaterials Annual Meeting and Exposition 2019: The Pinnacle of Biomaterials Innovation and Excellence - Transactions of the 42nd Annual Meeting. Society for Biomaterials, p. 971 1 p. (Transactions of the Annual Meeting of the Society for Biomaterials and the Annual International Biomaterials Symposium; vol. 40).
- X-ray phase contrast imaging of soft biomaterials in a whole-animal model Brown, J., Somo, S., Brooks, F., Komarov, S., Zhou, W., Anastasio, M. and Brey, E., 2019, Society for Biomaterials Annual Meeting and Exposition 2019: The Pinnacle of Biomaterials Innovation and Excellence - Transactions of the 42nd Annual Meeting. Society for Biomaterials, p. 971 1 p. (Transactions of the Annual Meeting of the Society for Biomaterials and the Annual International Biomaterials Symposium; vol. 40).
2018
- A 3D imaging system integrating photoacoustic and fluorescence orthogonal projections for anatomical, functional and molecular assessment of rodent models Brecht, H. P., Ivanov, V., Dumani, D. S., Emelianov, S. Y., Anastasio, M. A. and Ermilov, S. A., 2018, Photons Plus Ultrasound: Imaging and Sensing 2018. Wang, L. V. and Oraevsky, A. A. (eds.). SPIE, 1049411. (Progress in Biomedical Optics and Imaging - Proceedings of SPIE; vol. 10494).
- A deep Boltzmann machine-driven level set method for heart motion tracking using cine MRI images Wu, J., Mazur, T. R., Ruan, S., Lian, C., Daniel, N., Lashmett, H., Ochoa, L., Zoberi, I., Anastasio, M. A., Gach, H. M., Mutic, S., Thomas, M. and Li, H., Jul 2018, In: Medical Image Analysis. 47, p. 68-80 13 p.
- A diffuse optical tomography system for whole-brain functional imaging in mice using multiple camera views Markow, Z. E., Reisman, M. D., Bauer, A. Q., Eggebrecht, A. T., Anastasio, M. A. and Culver, J. P., 2018, Optical Tomography and Spectroscopy, OTS 2018. OSA - The Optical Society, (Optics InfoBase Conference Papers; vol. Part F90-OTS 2018).
- Active learning with noise modeling for medical image annotation Wu, J., Ruan, S., Lian, C., Mutic, S., Anastasio, M. A. and Li, H., May 23 2018, 2018 IEEE 15th International Symposium on Biomedical Imaging, ISBI 2018. IEEE Computer Society, p. 298-301 4 p. (Proceedings - International Symposium on Biomedical Imaging; vol. 2018-April).
- An advanced photoacoustic tomography system based on a ring geometry design Alshahrani, S., Yan, Y., Avrutsky, I., Malyarenko, E., Anastasio, M. and Mehrmohammadi, M., 2018, Medical Imaging 2018: Ultrasonic Imaging and Tomography. Duric, N. and Byram, B. C. (eds.). SPIE, 1058005. (Progress in Biomedical Optics and Imaging - Proceedings of SPIE; vol. 10580).
- Convolutional neural network based automatic plaque characterization for intracoronary optical coherence tomography images He, S., Zheng, J., Maehara, A., Mintz, G., Tang, D., Anastasio, M. and Li, H., 2018, Medical Imaging 2018: Image Processing. Angelini, E. D., Angelini, E. D. & Landman, B. A. (eds.). SPIE, 1057432. (Progress in Biomedical Optics and Imaging - Proceedings of SPIE; vol. 10574).
- First point-spread function and x-ray phase-contrast imaging results with an 88-mm diameter single crystal Lumpkin, A. H., Garson, A. B. and Anastasio, M. A., Jul 1 2018, In: Review of Scientific Instruments. 89, 7, 073704.
- Full-view 3D imaging system for functional and anatomical screening of the breast Oraevsky, A., Su, R., Nguyen, H., Moore, J., Lou, Y., Bhadra, S., Forte, L., Anastasio, M. and Yang, W., 2018, Photons Plus Ultrasound: Imaging and Sensing 2018. Wang, L. V. and Oraevsky, A. A. (eds.). SPIE, 104942Y. (Progress in Biomedical Optics and Imaging - Proceedings of SPIE; vol. 10494).
- Heart motion tracking on cine MRI based on a deep Boltzmann machine-driven level set method Wu, J., Ruan, S., Mazur, T. R., Daniel, N., Lashmett, H., Ochoa, L., Zoberi, I., Lian, C., Gach, H. M., Mutic, S., Thomas, M., Anastasio, M. A. and Li, H., May 23 2018, 2018 IEEE 15th International Symposium on Biomedical Imaging, ISBI 2018. IEEE Computer Society, p. 1153-1156 4 p. (Proceedings - International Symposium on Biomedical Imaging; vol. 2018-April).
- Improved two step reconstruction method in Ultrasound guided Diffuse Optical Tomography Shihab Uddin, K. M., Mostofa, A., Anastasio, M. and Zhu, Q., 2018, Optical Tomography and Spectroscopy, OTS 2018. OSA - The Optical Society, (Optics InfoBase Conference Papers; vol. Part F90-OTS 2018).
- Joint-reconstruction-enabled data acquisition design for single-shot edge-illumination x-ray phase-contrast tomography Chen, Y., Zhou, W. and Anastasio, M. A., 2018, Medical Imaging 2018: Physics of Medical Imaging. Schmidt, T. G., Chen, G.-H. and Lo, J. Y. (eds.). SPIE, 1057322. (Progress in Biomedical Optics and Imaging - Proceedings of SPIE; vol. 10573).
- Learning the ideal observer for SKE detection tasks by use of convolutional neural networks (Cum Laude Poster Award) Zhou, W. and Anastasio, M. A., 2018, Medical Imaging 2018: Image Perception, Observer Performance, and Technology Assessment. Samuelson, F. W. and Nishikawa, R. M. (eds.). SPIE, 1057719. (Progress in Biomedical Optics and Imaging - Proceedings of SPIE; vol. 10577).
- Learning-based stochastic object models for characterizing anatomical variations Dolly, S. R., Lou, Y., Anastasio, M. A. and Li, H., Mar 14 2018, In: Physics in medicine and biology. 63, 6, 065004.
- Parameterized joint reconstruction of the initial pressure and sound speed distributions for photoacoustic computed tomography Matthews, T. P., Poudel, J., Li, L., Wang, L. V. and Anastasio, M. A., 2018, In: SIAM Journal on Imaging Sciences. 11, 2, p. 1560-1588 29 p.
- Properties of a Joint Reconstruction Method for Edge-Illumination X-ray Phase-Contrast Tomography Chen, Y. and Anastasio, M. A., Dec 2018, In: Sensing and Imaging. 19, 1, 7.
- Subspace-based resolution-enhancing image reconstruction method for few-view differential phase-contrast tomography Guan, H., Hagen, C. K., Olivo, A. and Anastasio, M. A., Apr 1 2018, In: Journal of Medical Imaging. 5, 2, 023501.
- The Effectiveness of the Omnidirectional Illumination in Full-Ring Photoacoustic Tomography Alshahrani, S., Pattyn, A., Alijabbari, N., Yan, Y., Anastasio, M. and Mehrmohammadi, M., Dec 17 2018, 2018 IEEE International Ultrasonics Symposium, IUS 2018. IEEE Computer Society, 8580099. (IEEE International Ultrasonics Symposium, IUS; vol. 2018-October).
2017
- A forward-adjoint operator pair based on the elastic wave equation for use in transcranial photoacoustic computed tomography Mitsuhashi, K., Poudel, J., Matthews, T. P., Garcia-Uribe, A., Wang, L. V. and Anastasio, M. A., 2017, In: SIAM Journal on Imaging Sciences. 10, 4, p. 2022-2048 27 p.
- A joint-reconstruction approach for single-shot edge illumination x-ray phase-contrast tomography Chen, Y., Guan, H., Hagen, C. K., Olivo, A. and Anastasio, M. A., 2017, Medical Imaging 2017: Physics of Medical Imaging. Schmidt, T. G., Lo, J. Y. and Flohr, T. G. (eds.). SPIE, 1013217. (Progress in Biomedical Optics and Imaging - Proceedings of SPIE; vol. 10132).
- A resolution-enhancing image reconstruction method for few-view differential phase-contrast tomography Guan, H. and Anastasio, M. A., 2017, Medical Imaging 2017: Physics of Medical Imaging. Schmidt, T. G., Lo, J. Y. and Flohr, T. G. (eds.). SPIE, 1013216. (Progress in Biomedical Optics and Imaging - Proceedings of SPIE; vol. 10132).
- Design and development of a full-ring ultrasound and photoacoustic tomography system for breast cancer imaging: Design and development of a full-ring ultrasound and photoacoustic tomography system for breast cancer imaging Alshahrani, S., Yan, Y., Avrutsky, I., Anastasio, M., Malyarenko, E., Duric, N. and Mehrmohammadi, M., Oct 31 2017, 2017 IEEE International Ultrasonics Symposium, IUS 2017. IEEE Computer Society, 8092129. (IEEE International Ultrasonics Symposium, IUS).
- Estimating posterior image variance with sparsity-based object priors for MRI Chen, Y., Lou, Y., Eldeniz, C., An, H. and Anastasio, M. A., 2017, Medical Imaging 2017: Physics of Medical Imaging. Schmidt, T. G., Lo, J. Y. & Flohr, T. G. (eds.). SPIE, 101321J. (Progress in Biomedical Optics and Imaging - Proceedings of SPIE; vol. 10132).
- Generation of anatomically realistic numerical phantoms for photoacoustic and ultrasonic breast imaging Lou, Y., Zhou, W., Matthews, T. P., Appleton, C. M. and Anastasioa, M. A., Apr 1 2017, In: Journal of biomedical optics. 22, 4, 041015.
- Half-time image reconstruction in photoacoustic tomography Anastasio, M. A., Zhang, J., Pan, X. and Wang, L. V., Jan 1 2017, Photoacoustic Imaging and Spectroscopy. CRC Press, p. 155-163 9 p.
- Image reconstruction for ultrasound computed tomography by use of the regularized dual averaging method Matthews, T. P., Wang, K., Li, C., Duric, N. and Anastasio, M. A., 2017, Medical Imaging 2017: Ultrasonic Imaging and Tomography. Duric, N., Duric, N. & Heyde, B. (eds.). SPIE, 101390P. (Progress in Biomedical Optics and Imaging - Proceedings of SPIE; vol. 10139).
- Image reconstruction in optoacoustic tomography accounting for frequency-dependent attenuation La Rivière, P., Zhang, J. and Anastasio, M. A., Jan 1 2017, Photoacoustic Imaging and Spectroscopy. CRC Press, p. 145-154 10 p.
- Iterative image reconstruction in elastic inhomogenous media with application to transcranial photoacoustic tomography Poudel, J., Matthews, T. P., Mitsuhashi, K., Garcia-Uribe, A., Wang, L. V. and Anastasio, M. A., 2017, Medical Imaging 2017: Ultrasonic Imaging and Tomography. Duric, N., Duric, N. and Heyde, B. (eds.). SPIE, 101390C. (Progress in Biomedical Optics and Imaging - Proceedings of SPIE; vol. 10139).
- Joint reconstruction of the initial pressure and speed of sound distributions from combined photoacoustic and ultrasound tomography measurements Matthews, T. P. and Anastasio, M. A., Nov 8 2017, In: Inverse Problems. 33, 12, 124002.
- Joint reconstruction of the sound speed and initial pressure distributions for ultrasound computed tomography and photoacoustic computed tomography Matthews, T. P. and Anastasio, M. A., 2017, Medical Imaging 2017: Ultrasonic Imaging and Tomography. Duric, N., Duric, N. and Heyde, B. (eds.). SPIE, 101390B. (Progress in Biomedical Optics and Imaging - Proceedings of SPIE; vol. 10139).
- Learning-based stochastic object models for use in optimizing imaging systems Dolly, S. R., Anastasio, M. A., Yu, L. and Li, H., 2017, Medical Imaging 2017: Physics of Medical Imaging. Schmidt, T. G., Lo, J. Y. and Flohr, T. G. (eds.). SPIE, 101321T. (Progress in Biomedical Optics and Imaging - Proceedings of SPIE; vol. 10132).
- Mitigation of artifacts due to isolated acoustic heterogeneities in photoacoustic computed tomography using a variable data truncation-based reconstruction method Poudel, J., Matthews, T. P., Li, L., Anastasio, M. A. and Wang, L. V., Apr 1 2017, In: Journal of biomedical optics. 22, 4, 041018.
- Recent advances in edge illumination x-ray phase-contrast tomography Zamir, A., Hagen, C., Diemoz, P. C., Endrizzi, M., Vittoria, F., Chen, Y., Anastasio, M. A. and Olivo, A., Oct 1 2017, In: Journal of Medical Imaging. 4, 4, 040901.
- Regularized Dual Averaging Image Reconstruction for Full-Wave Ultrasound Computed Tomography Matthews, T. P., Wang, K., Li, C., Duric, N. and Anastasio, M. A., May 2017, In: IEEE Transactions on Ultrasonics, Ferroelectrics, and Frequency Control. 64, 5, p. 811-825 15 p., 7878669.
- Single-shot edge illumination x-ray phase-contrast tomography enabled by joint image reconstruction Chen, Y., Guan, H., Hagen, C. K., Olivo, A. and Anastasio, M. A., Feb 1 2017, In: Optics Letters. 42, 3, p. 619-622 4 p.
- Special section guest editorial: Photoacoustic imaging and sensing Anastasio, M. A., Beard, P. C. and Zhu, Q., Apr 1 2017, In: Journal of biomedical optics. 22, 4, 041001.
- Task-based data-acquisition optimization for sparse image reconstruction systems Chen, Y., Lou, Y., Kupinski, M. A. and Anastasio, M. A., 2017, Medical Imaging 2017: Image Perception, Observer Performance, and Technology Assessment. Nishikawa, R. M. & Kupinski, M. A. (eds.). SPIE, 101360Z. (Progress in Biomedical Optics and Imaging - Proceedings of SPIE; vol. 10136).
- Task-based image quality assessment in radiation therapy: Initial characterization and demonstration with CT simulation images Dolly, S. R., Anastasio, M. A., Yu, L. and Li, H., 2017, Medical Imaging 2017: Image Perception, Observer Performance, and Technology Assessment. Nishikawa, R. M. and Kupinski, M. A. (eds.). SPIE, 101360Y. (Progress in Biomedical Optics and Imaging - Proceedings of SPIE; vol. 10136).
- Two step imaging reconstruction using truncated pseudoinverse as a preliminary estimate in ultrasound guided diffuse optical tomography Shihab Uddin, K. M., Mostafa, A., Anastasio, M. and Zhu, Q., Dec 1 2017, In: Biomedical Optics Express. 8, 12, p. 5437-5449 13 p., #304054.
2016
- A comparative study based on image quality and clinical task performance for CT reconstruction algorithms in radiotherapy Li, H., Dolly, S., Chen, H. C., Anastasio, M. A., Low, D. A., Li, H. H., Michalski, J. M., Thorstad, W. L., Gay, H. and Mutic, S., 2016, In: Journal of Applied Clinical Medical Physics. 17, 4, p. 377-390 14 p.
- Accelerated fast iterative shrinkage thresholding algorithms for sparsity-regularized cone-beam CT image reconstruction Xu, Q., Yang, D., Tan, J., Sawatzky, A. and Anastasio, M. A., 2016, In: Medical Physics. 43, 4, p. 1849-1872 24 p.
- Advanced laser system for 3D optoacoustic tomography of the breast Klosner, M., Chan, G., Wu, C., Heller, D. F., Su, R., Ermilov, S., Brecht, H. P., Ivanov, V., Talole, P., Lou, Y., Anastasio, M. and Oraevsky, A., 2016, Photons Plus Ultrasound: Imaging and Sensing 2016. Oraevsky, A. A. and Wang, L. V. (eds.). SPIE, 97085B. (Progress in Biomedical Optics and Imaging - Proceedings of SPIE; vol. 9708).
- An integrated model-driven method for in-treatment upper airway motion tracking using cine MRI in head and neck radiation therapy Li, H., Chen, H. C., Dolly, S., Li, H., Fischer-Valuck, B., Victoria, J., Dempsey, J., Ruan, S., Anastasio, M., Mazur, T., Gach, M., Kashani, R., Green, O., Rodriguez, V., Gay, H., Thorstad, W. and Mutic, S., Aug 1 2016, In: Medical Physics. 43, 8, p. 4700-4710 11 p.
- Application of signal detection theory to assess optoacoustic imaging systems Lou, Y., Oraevsky, A. and Anastasio, M. A., 2016, Photons Plus Ultrasound: Imaging and Sensing 2016. Oraevsky, A. A. and Wang, L. V. (eds.). SPIE, 97083Z. (Progress in Biomedical Optics and Imaging - Proceedings of SPIE; vol. 9708).
- Compensation for acoustic heterogeneities in photoacoustic computed tomography using a variable temporal data truncation reconstruction method Poudel, J., Matthews, T. P., Anastasio, M. A. and Wang, L. V., 2016, Photons Plus Ultrasound: Imaging and Sensing 2016. Oraevsky, A. A. & Wang, L. V. (eds.). SPIE, 97083W. (Progress in Biomedical Optics and Imaging - Proceedings of SPIE; vol. 9708).
- Compensation for air voids in photoacoustic computed tomography image reconstruction Matthews, T. P., Li, L., Wang, L. V. and Anastasio, M. A., 2016, Photons Plus Ultrasound: Imaging and Sensing 2016. Oraevsky, A. A. and Wang, L. V. (eds.). SPIE, 970841. (Progress in Biomedical Optics and Imaging - Proceedings of SPIE; vol. 9708).
- Evaluation of the tissue response to alginate encapsulated islets in an omentum pouch model: Evaluation of the tissue response to alginate encapsulated islets in an omentum pouch model Ibarra, V., Appel, A. A., Anastasio, M. A., Opara, E. C. and Brey, E. M., Jul 1 2016, In: Journal of Biomedical Materials Research - Part A. 104, 7, p. 1581-1590 10 p.
- Generation of anatomically realistic numerical phantoms for optoacoustic breast imaging Lou, Y., Mitsuhashi, K., Appleton, C. M., Oraevsky, A. and Anastasio, M. A., 2016, Photons Plus Ultrasound: Imaging and Sensing 2016. Oraevsky, A. A. and Wang, L. V. (eds.). SPIE, 97084O. (Progress in Biomedical Optics and Imaging - Proceedings of SPIE; vol. 9708).
- Imaging of Hydrogel Microsphere Structure and Foreign Body Response Based on Endogenous X-Ray Phase Contrast Appel, A. A., Ibarra, V., Somo, S. I., Larson, J. C., Garson, A. B., Guan, H., McQuilling, J. P., Zhong, Z., Anastasio, M. A., Opara, E. C. and Brey, E. M., Nov 2016, In: Tissue Engineering - Part C: Methods. 22, 11, p. 1038-1048 11 p.
- Impact of nonstationary optical illumination on image reconstruction in optoacoustic tomography Lou, Y., Wang, K., Oraevsky, A. A. and Anastasio, M. A., Dec 1 2016, In: Journal of the Optical Society of America A: Optics and Image Science, and Vision. 33, 12, p. 2333-2347 15 p.
- In vivo small animal lung speckle imaging with a benchtop in-line XPC system Garson, A. B., Gunsten, S., Vasireddi, S., Brody, S. and Anastasio, M. A., 2016, Medical Imaging 2016: Physics of Medical Imaging. Kontos, D., Lo, J. Y. and Flohr, T. G. (eds.). SPIE, 97834Z. (Progress in Biomedical Optics and Imaging - Proceedings of SPIE; vol. 9783).
- Joint Reconstruction of Absorbed Optical Energy Density and Sound Speed Distributions in Photoacoustic Computed Tomography: A Numerical Investigation Huang, C., Wang, K., Schoonover, R. W., Wang, L. V. and Anastasio, M. A., Jun 2016, In: IEEE Transactions on Computational Imaging. 2, 2, p. 136-149 14 p., 7394148.
- Joint reconstruction of absorption and refractive properties in propagation-based x-ray phase-contrast tomography via a non-linear image reconstruction algorithm Chen, Y., Wang, K., Gursoy, D., Soriano, C., De Carlo, F. and Anastasio, M. A., 2016, Medical Imaging 2016: Physics of Medical Imaging. Kontos, D., Lo, J. Y. and Flohr, T. G. (eds.). SPIE, 97835H. (Progress in Biomedical Optics and Imaging - Proceedings of SPIE; vol. 9783).
- Label-free photoacoustic tomography of whole mouse brain structures ex vivo Li, L., Xia, J., Li, G., Garcia-Uribe, A., Sheng, Q., Anastasio, M. A. and Wanga, L. V., Jul 1 2016, In: Neurophotonics. 3, 3, 035001.
- Practical considerations for noise power spectra estimation for clinical CT scanners Dolly, S., Chen, H. C., Anastasio, M., Mutic, S. and Li, H., 2016, In: Journal of Applied Clinical Medical Physics. 17, 3, p. 392-407 16 p.
- Quantification of signal detection performance degradation induced by phase-retrieval in propagation-based x-ray phase-contrast imaging Chou, C. Y. and Anastasio, M. A., 2016, Medical Imaging 2016: Physics of Medical Imaging. Kontos, D., Lo, J. Y. & Flohr, T. G. (eds.). SPIE, 97835J. (Progress in Biomedical Optics and Imaging - Proceedings of SPIE; vol. 9783).
- Three-dimensional optoacoustic and laser-induced ultrasound tomography system for preclinical research in mice: Design and phantom validation Ermilov, S. A., Su, R., Conjusteau, A., Anis, F., Nadvoretskiy, V., Anastasio, M. A. and Oraevsky, A. A., Jan 1 2016, In: Ultrasonic Imaging. 38, 1, p. 77-95 19 p.
- X-ray Phase Contrast Allows Three Dimensional, Quantitative Imaging of Hydrogel Implants Appel, A. A., Larson, J. C., Jiang, B., Zhong, Z., Anastasio, M. A. and Brey, E. M., Mar 1 2016, In: Annals of Biomedical Engineering. 44, 3, p. 773-781 9 p.
2015
- A Constrained Variable Projection Reconstruction Method for Photoacoustic Computed Tomography Without Accurate Knowledge of Transducer Responses Sheng, Q., Wang, K., Matthews, T. P., Xia, J., Zhu, L., Wang, L. V. and Anastasio, M. A., Dec 2015, In: IEEE transactions on medical imaging. 34, 12, p. 2443-2458 16 p., 7113884.
- Accelerated iterative image reconstruction in three-dimensional optoacoustic tomography Anis, F., Wang, K., Su, R., Ermilov, S. A., Oraevsky, A. A. and Anastasio, M. A., 2015, Photons Plus Ultrasound: Imaging and Sensing 2015. Oraevsky, A. A. & Wang, L. V. (eds.). SPIE, 932315. (Progress in Biomedical Optics and Imaging - Proceedings of SPIE; vol. 9323).
- Automated contouring error detection based on supervised geometric attribute distribution models for radiation therapy: A general strategy Chen, H. C., Tan, J., Dolly, S., Kavanaugh, J., Anastasio, M. A., Low, D. A., Harold Li, H., Altman, M., Gay, H., Thorstad, W. L., Mutic, S. and Li, H., Feb 1 2015, In: Medical Physics. 42, 2, p. 1048-1059 12 p.
- Boundary-enhancement in propagation-based x-ray phase-contrast tomosynthesis improves depth position characterization Guan, H., Xu, Q., Garson, A. B. and Anastasio, M. A., Apr 21 2015, In: Physics in medicine and biology. 60, 8, p. N151-N165 N151.
- Breast ultrasound computed tomography using waveform inversion with source encoding Wang, K., Matthews, T., Anis, F., Li, C., Duric, N. and Anastasio, M. A., 2015, Medical Imaging 2015: Ultrasonic Imaging and Tomography. Duric, N. & Bosch, J. G. (eds.). SPIE, 94190C. (Progress in Biomedical Optics and Imaging - Proceedings of SPIE; vol. 9419).
- Effect of rotating partial illumination on image reconstruction for optoacoustic breast tomography Lou, Y., Nadvoretskiy, V., Wang, K., Ermilov, S., Oraevsky, A. and Anastasio, M. A., 2015, Photons Plus Ultrasound: Imaging and Sensing 2015. Oraevsky, A. A. and Wang, L. V. (eds.). SPIE, 93233L. (Progress in Biomedical Optics and Imaging - Proceedings of SPIE; vol. 9323).
- Image reconstruction in transcranial photoacoustic computed tomography of the brain Mitsuhashi, K., Wang, L. V. and Anastasio, M. A., 2015, Photons Plus Ultrasound: Imaging and Sensing 2015. Oraevsky, A. A. and Wang, L. V. (eds.). SPIE, 93233B. (Progress in Biomedical Optics and Imaging - Proceedings of SPIE; vol. 9323).
- Photoacoustic and thermoacoustic tomography: Image formation principles Wang, K. and Anastasio, M. A., Jan 1 2015, Handbook of Mathematical Methods in Imaging: Volume 1, Second Edition. Springer, p. 1081-1116 36 p.
- Photoacoustic computed tomography without accurate ultrasonic transducer responses Sheng, Q., Wang, K., Xia, J., Zhu, L., Wang, L. V. and Anastasio, M. A., 2015, Photons Plus Ultrasound: Imaging and Sensing 2015. Oraevsky, A. A. and Wang, L. V. (eds.). SPIE, 932313. (Progress in Biomedical Optics and Imaging - Proceedings of SPIE; vol. 9323).
- Small animal lung imaging with an in-line X-ray phase contrast benchtop system Garson, A. B., Gunsten, S., Guan, H., Vasireddi, S., Brody, S. and Anastasio, M. A., 2015, Medical Imaging 2015: Physics of Medical Imaging. Hoeschen, C., Kontos, D. and Hoeschen, C. (eds.). SPIE, 94120L. (Progress in Biomedical Optics and Imaging - Proceedings of SPIE; vol. 9412).
- Sparsity-driven ideal observer for computed medical imaging systems Wang, K., Lou, Y., Kupinski, M. A. and Anastasio, M. A., 2015, Medical Imaging 2015: Image Perception, Observer Performance, and Technology Assessment. Mello-Thoms, C. R., Kupinski, M. A. and Mello-Thoms, C. R. (eds.). SPIE, 94161C. (Progress in Biomedical Optics and Imaging - Proceedings of SPIE; vol. 9416).
- Synergistic image reconstruction for hybrid ultrasound and photoacoustic computed tomography Matthews, T. P., Wang, K., Wang, L. V. and Anastasio, M. A., 2015, Photons Plus Ultrasound: Imaging and Sensing 2015. Oraevsky, A. A. and Wang, L. V. (eds.). SPIE, 93233A. (Progress in Biomedical Optics and Imaging - Proceedings of SPIE; vol. 9323).
- Three-dimensional laser optoacoustic and laser ultrasound imaging system for biomedical research Ermilov, S. A., Su, R., Conjusteau, A., Oruganti, T., Wang, K., Anis, F., Anastasio, M. A. and Oraevsky, A. A., 2015, Photons Plus Ultrasound: Imaging and Sensing 2015. Oraevsky, A. A. and Wang, L. V. (eds.). SPIE, 93230N. (Progress in Biomedical Optics and Imaging - Proceedings of SPIE; vol. 9323).
- Waveform inversion with source encoding for breast sound speed reconstruction in ultrasound computed tomography Wang, K., Matthews, T., Anis, F., Li, C., Duric, N. and Anastasio, M., Mar 2015, In: IEEE Transactions on Ultrasonics, Ferroelectrics, and Frequency Control. 62, 3, p. 475-493 19 p., 7055442.
- X-ray phase contrast imaging of calcified tissue and biomaterial structure in bioreactor engineered tissues Appel, A. A., Larson, J. C., Garson, A. B., Guan, H., Zhong, Z., Nguyen, B. N. B., Fisher, J. P., Anastasio, M. A. and Brey, E. M., Mar 1 2015, In: Biotechnology and bioengineering. 112, 3, p. 612-620 9 p.
2014
- 3D laser optoacoustic ultrasonic imaging system for research in mice (LOUIS-3DM) Ermilov, S. A., Su, R., Conjusteau, A., Ivanov, V., Nadvoretskiy, V., Oruganti, T., Talole, P., Anis, F., Anastasio, M. A. and Oraevsky, A. A., 2014, Photons Plus Ultrasound: Imaging and Sensing 2014. SPIE, 89430J. (Progress in Biomedical Optics and Imaging - Proceedings of SPIE; vol. 8943).
- A multi-channel image reconstruction method for grating-based X-ray phase-contrast computed Tomography Xu, Q., Sawatzky, A. and Anastasio, M. A., 2014, Medical Imaging 2014: Physics of Medical Imaging. SPIE, 90330D. (Progress in Biomedical Optics and Imaging - Proceedings of SPIE; vol. 9033).
- Acoustic-speed correction of photoacoustic tomography by ultrasonic computed tomography based on optical excitation of elements of a full-ring transducer array Xia, J., Huang, C., Maslov, K., Anastasio, M. A. and Wang, L. V., 2014, Photons Plus Ultrasound: Imaging and Sensing 2014. SPIE, 89432G. (Progress in Biomedical Optics and Imaging - Proceedings of SPIE; vol. 8943).
- Automatic CT simulation optimization for radiation therapy: A general strategy Li, H., Yu, L., Anastasio, M. A., Chen, H. C., Tan, J., Gay, H., Michalski, J. M., Low, D. A. and Mutic, S., Mar 2014, In: Medical Physics. 41, 3, 031913.
- Depth resolution properties of in-line X-ray phase-contrast Tomosynthesis Guan, H., Xu, Q., Garson, A. and Anastasio, M. A., 2014, Medical Imaging 2014: Physics of Medical Imaging. SPIE, 90330H. (Progress in Biomedical Optics and Imaging - Proceedings of SPIE; vol. 9033).
- Discrete imaging models for three-dimensional optoacoustic tomography using radially symmetric expansion functions Wang, K., Schoonover, R. W., Su, R., Oraevsky, A. and Anastasio, M. A., May 2014, In: IEEE Transactions on Medical Imaging. 33, 5, p. 1180-1193 14 p., 6749001.
- Fast spatiotemporal image reconstruction based on low-rank matrix estimation for dynamic photoacoustic computed tomography Wang, K., Xia, J., Li, C., Wang, L. V. and Anastasio, M. A., May 2014, In: Journal of biomedical optics. 19, 5, 056007.
- In-line X-ray phase-contrast lung imaging in situ with a benchtop system Garson, A. B., Izaguirre, E. W., Price, S. G., Guan, H., Vasireddi, S. K. and Anastasio, M. A., 2014, Medical Imaging 2014: Physics of Medical Imaging. SPIE, 90330A. (Progress in Biomedical Optics and Imaging - Proceedings of SPIE; vol. 9033).
- Investigation of effective system designs for transcranial photoacoustic tomography of the brain Mitsuhashi, K., Schoonover, R. W., Huang, C., Wang, L. V. and Anastasio, M. A., 2014, Photons Plus Ultrasound: Imaging and Sensing 2014. SPIE, 894369. (Progress in Biomedical Optics and Imaging - Proceedings of SPIE; vol. 8943).
- Investigation of the adjoint-state method for ultrasound computed tomography: A numerical and experimental study Anis, F., Lou, Y., Conjusteau, A., Su, R., Oruganti, T., Ermilov, S. A., Oraevsky, A. A. and Anastasio, M. A., 2014, Photons Plus Ultrasound: Imaging and Sensing 2014. SPIE, 894337. (Progress in Biomedical Optics and Imaging - Proceedings of SPIE; vol. 8943).
- Investigation of the far-field approximation for modeling a transducer's spatial impulse response in photoacoustic computed tomography Mitsuhashi, K., Wang, K. and Anastasio, M. A., Mar 2014, In: Photoacoustics. 2, 1, p. 21-32 12 p.
- Low-rank matrix estimation-based spatio-temporal image reconstruction for dynamic photoacoustic computed tomography Wang, K., Xia, J., Li, C., Wang, L. V. and Anastasio, M. A., 2014, Photons Plus Ultrasound: Imaging and Sensing 2014. SPIE, 89432I. (Progress in Biomedical Optics and Imaging - Proceedings of SPIE; vol. 8943).
- Proximal ADMM for multi-channel image reconstruction in spectral X-ray CT Sawatzky, A., Xu, Q., Schirra, C. O. and Anastasio, M. A., Aug 2014, In: IEEE Transactions on Medical Imaging. 33, 8, p. 1637-1668 32 p., 6808543.
- Retrospective respiration-gated whole-body photoacoustic computed tomography of mice Xia, J., Chen, W., Maslov, K., Anastasio, M. A. and Wang, L. V., Jan 2014, In: Journal of biomedical optics. 19, 1, 016003.
- Simultaneous reconstruction of absorbed optical energy density and speed of sound distributions in photoacoustic computed tomography Huang, C., Wang, K., Schoonover, R. W., Wang, L. V. and Anastasio, M. A., 2014, Photons Plus Ultrasound: Imaging and Sensing 2014. SPIE, 894360. (Progress in Biomedical Optics and Imaging - Proceedings of SPIE; vol. 8943).
- Sparsity-regularized image reconstruction of decomposed K-edge data in spectral CT Xu, Q., Sawatzky, A., Anastasio, M. A. and Schirra, C. O., May 21 2014, In: Physics in medicine and biology. 59, 10, p. N65-N79
- Spectral CT: A technology primer for contrast agent development Schirra, C. O., Brendel, B., Anastasio, M. A. and Roessl, E., Jan 2014, In: Contrast Media and Molecular Imaging. 9, 1, p. 62-70 9 p.
2013
- 3D laser optoacoustic ultrasonic imaging system for preclinical research Ermilov, S. A., Conjusteau, A., Hernandez, T., Su, R., Nadvoretskiy, V., Tsyboulski, D., Anis, F., Anastasio, M. A. and Oraevsky, A. A., May 28 2013, Photons Plus Ultrasound: Imaging and Sensing 2013. 85810N. (Progress in Biomedical Optics and Imaging - Proceedings of SPIE; vol. 8581).
- A simple Fourier transform-based reconstruction formula for photoacoustic computed tomography with a circular or spherical measurement geometry Wang, K. and Anastasio, M. A., May 28 2013, Photons Plus Ultrasound: Imaging and Sensing 2013. 85814K. (Progress in Biomedical Optics and Imaging - Proceedings of SPIE; vol. 8581).
- Accelerating image reconstruction in three-dimensional optoacoustic tomography on graphics processing units Wang, K., Huang, C., Kao, Y. J., Chou, C. Y., Oraevsky, A. A. and Anastasio, M. A., Feb 2013, In: Medical Physics. 40, 2, 023301.
- An initial evaluation of analyser-based phase-contrast X-ray imaging of carotid plaque microstructure Appel, A. A., Chou, C. Y., Larson, J. C., Zhong, Z., Schoen, F. J., Johnston, C. M., Brey, E. M. and Anastasio, M. A., Jan 1 2013, In: British Journal of Radiology. 86, 1021, 20120318.
- Characterization of speckle in lung images acquired with a benchtop in-line x-ray phase-contrast system Garson, A. B., Izaguirre, E. W., Price, S. G. and Anastasio, M. A., Jun 21 2013, In: Physics in medicine and biology. 58, 12, p. 4237-4253 17 p.
- Enhancement of photoacoustic tomography by ultrasonic computed tomography based on optical excitation of elements of a full-ring transducer array Xia, J., Huang, C., Maslov, K., Anastasio, M. A. and Wang, L. V., 2013, In: Optics Letters. 38, 16, p. 3140-3143 4 p.
- Full-wave iterative image reconstruction in photoacoustic tomography with acoustically inhomogeneous media Huang, C., Wang, K., Nie, L., Wang, L. V. and Anastasio, M. A., 2013, In: IEEE transactions on medical imaging. 32, 6, p. 1097-1110 14 p., 6484982.
- Image reconstruction and system optimization for three-dimensional speed of sound tomography using laser-induced ultrasound Anis, F., Su, R., Nadvoretsky, V. V., Conjusteau, A., Ermilov, S. A., Oraevsky, A. A. and Anastasio, M. A., May 28 2013, Photons Plus Ultrasound: Imaging and Sensing 2013. 85814T. (Progress in Biomedical Optics and Imaging - Proceedings of SPIE; vol. 8581).
- Image reconstruction in photoacoustic tomography with heterogeneous media using an iterative method Huang, C., Wang, K., Wang, L. V. and Anastasio, M. A., 2013, Photons Plus Ultrasound: Imaging and Sensing 2013. 858106. (Progress in Biomedical Optics and Imaging - Proceedings of SPIE; vol. 8581).
- Imaging challenges in biomaterials and tissue engineering Appel, A. A., Anastasio, M. A., Larson, J. C. and Brey, E. M., Sep 2013, In: Biomaterials. 34, 28, p. 6615-6630 16 p.
- Iterative image reconstruction in photoacoustic tomography using Kaiser-Bessel windows Schoonover, R. W., Wang, K. and Anastasio, M. A., May 28 2013, Photons Plus Ultrasound: Imaging and Sensing 2013. 85814X. (Progress in Biomedical Optics and Imaging - Proceedings of SPIE; vol. 8581).
- Principles and applications of photoacoustic computed tomography Anis, F., Wang, K. and Anastasio, M. A., Oct 1 2013, Molecular Imaging Techniques: New Frontiers. Future Medicine Ltd., p. 129-147 19 p.
- Statistical reconstruction of material decomposed data in spectral CT Schirra, C. O., Roessl, E., Koehler, T., Brendel, B., Thran, A., Pan, D., Anastasio, M. A. and Proksa, R., 2013, In: IEEE transactions on medical imaging. 32, 7, p. 1249-1257 9 p., 6473891.
2012
- A simple Fourier transform-based reconstruction formula for photoacoustic computed tomography with a circular or spherical measurement geometry Wang, K. and Anastasio, M. A., Dec 7 2012, In: Physics in medicine and biology. 57, 23, p. N493-N499
- Aberration correction for transcranial photoacoustic tomography of primates employing adjunct image data Huang, C., Nie, L., Schoonover, R. W., Guo, Z., Schirra, C. O., Anastasio, M. A. and Wang, L. V., Jun 2012, In: Journal of biomedical optics. 17, 6, 066016.
- Adaptation of a clustered lumpy background model for task-based image quality assessment in x-ray phase-contrast mammography Zysk, A. M., Brankov, J. G., Wernick, M. N. and Anastasio, M. A., Feb 2012, In: Medical Physics. 39, 2, p. 906-911 6 p.
- Analysis of the role of shear waves in transcranial photoacoustic tomography with a planar geometry Schoonover, R. W., Wang, L. V. and Anastasio, M. A., 2012, Photons Plus Ultrasound: Imaging and Sensing 2012. SPIE, 822320. (Progress in Biomedical Optics and Imaging - Proceedings of SPIE; vol. 8223).
- Analyzer-based phase-contrast x-ray imaging of carotid plaque microstructure Appel, A. A., Chou, C. Y., Greisler, H. P., Larson, J. C., Vasireddi, S., Zhong, Z., Anastasio, M. A. and Brey, E. M., Nov 2012, In: American Journal of Surgery. 204, 5, p. 631-636 6 p.
- Compensation of shear waves in photoacoustic tomography with layered acoustic media Schoonover, R. W. and Anastasio, M. A., Apr 9 2012, Photons Plus Ultrasound: Imaging and Sensing 2012. 82232P. (Progress in Biomedical Optics and Imaging - Proceedings of SPIE; vol. 8223).
- Emerging imaging technologies in medicine Anastasio, M. A. and La Riviere, P., Jan 1 2012, CRC Press. 353 p.
- Framework for computing the spatial coherence effects of polycapillary x-ray optics Zysk, A. M., Schoonover, R. W., Xu, Q. and Anastasio, M. A., Feb 13 2012, In: Optics Express. 20, 4, p. 3975-3982 8 p.
- Imaging of poly(a-hydroxy-ester) scaffolds with X-ray phase-contrast microcomputed tomography Appel, A. A., Larson, J. C., Somo, S., Zhong, Z., Spicer, P. P., Kasper, F. K., Garson, A. B., Zysk, A. M., Mikos, A. G., Anastasio, M. A. and Brey, E. M., Nov 1 2012, In: Tissue Engineering - Part C: Methods. 18, 11, p. 859-865 7 p.
- Investigation of discrete imaging models and iterative image reconstruction in differential X-ray phase-contrast tomography Xu, Q., Sidky, E. Y., Pan, X., Stampanoni, M., Modregger, P. and Anastasio, M. A., May 7 2012, In: Optics Express. 20, 10, p. 10724-10749 26 p.
- Investigation of iterative image reconstruction in optoacoustic tomography Wang, K., Su, R., Oraevsky, A. A. and Anastasio, M. A., Apr 9 2012, Photons Plus Ultrasound: Imaging and Sensing 2012. 82231Y. (Progress in Biomedical Optics and Imaging - Proceedings of SPIE; vol. 8223).
- Investigation of iterative image reconstruction in three-dimensional optoacoustic tomography Wang, K., Su, R., Oraevsky, A. A. and Anastasio, M. A., Sep 7 2012, In: Physics in medicine and biology. 57, 17, p. 5399-5423 25 p.
- Nondestructive volumetric imaging of tissue microstructure with benchtop x-ray phase-contrast tomography and critical point drying Zysk, A. M., Garson, A. B., Xu, Q., Brey, E. M., Zhou, W., Brankov, J. G., Wernick, M. N., Kuszak, J. R. and Anastasio, M. A., Aug 1 2012, In: Biomedical Optics Express. 3, 8, p. 1924-1932 9 p.
- Numerical investigation of the effects of shear waves in transcranial photoacoustic tomography with a planar geometry. Schoonover, R. W., Wang, L. V. and Anastasio, M. A., Jun 2012, In: Journal of biomedical optics. 17, 6, p. 61215 1 p.
- Photoacoustic computed tomography correcting for heterogeneity and attenuation Huang, C., Nie, L., Schoonover, R. W., Wang, L. V. and Anastasio, M. A., Jun 2012, In: Journal of biomedical optics. 17, 6, 061211.
- Photoacoustic imaging and sensing Anastasio, M. A. and Beard, P. C., Jun 2012, In: Journal of biomedical optics. 17, 6, 061201.
- Photoacoustic tomography through a whole adult human skull with a photon recycler Nie, L., Cai, X., Maslov, K., Garcia-Uribe, A., Anastasio, M. A. and Wang, L. V., Nov 2012, In: Journal of biomedical optics. 17, 11, 110506.
- Rapid propeller-MRI: A combination of iterative reconstruction and under-sampling Tamhane, A. A., Arfanakis, K., Anastasio, M., Guo, X., Vannier, M. and Gao, J. H., Nov 2012, In: Journal of Magnetic Resonance Imaging. 36, 5, p. 1241-1247 7 p.
- Ring-shaped confocal photoacoustic computed tomography for small-animal whole-body imaging Xia, J., Chatni, M., Maslov, K., Guo, Z., Anastasio, M. and Wang, L. V., 2012, Photons Plus Ultrasound: Imaging and Sensing 2012. SPIE, 82230G. (Progress in Biomedical Optics and Imaging - Proceedings of SPIE; vol. 8223).
- Ring-shaped confocal photoacoustic computed tomography for small-animal whole-body imaging Xia, J., Chatni, M., Maslov, K., Guo, Z., Sohn, R., Arbeit, J., Anastasio, M., Zhu, Q. and Wang, L. V., 2012, Biomedical Optics, BIOMED 2012. Optical Society of America (OSA), p. BSu3A.43 (Biomedical Optics, BIOMED 2012).
- Sparsity regularized data-space restoration in optoacoustic tomography Wang, K., Su, R., Oraevsky, A. A. and Anastasio, M. A., Apr 9 2012, Photons Plus Ultrasound: Imaging and Sensing 2012. 822322. (Progress in Biomedical Optics and Imaging - Proceedings of SPIE; vol. 8223).
- SU-F-BRCD-09: Total Variation (TV) Based Fast Convergent Iterative CBCT Reconstruction with GPU Acceleration xu, Q., Yang, D., Tan, J. and Anastasio, M., Jun 2012, In: Medical Physics. 39, 6, p. 3857 1 p.
- Transcranial photoacoustic tomography of the monkey brain Nie, L., Huang, C., Guo, Z., Anastasio, M. and Wang, L. V., 2012, Photons Plus Ultrasound: Imaging and Sensing 2012. 82230L. (Progress in Biomedical Optics and Imaging - Proceedings of SPIE; vol. 8223).
- Transducer field imaging using acoustography Sandhu, J. S., Schoonover, R. W., Weber, J. I., Tawiah, J., Kunin, V. and Anastasio, M. A., Jul 16 2012, In: Advances in Acoustics and Vibration. 275858.
- Tumor glucose metabolism imaged in vivoin small animals with whole-body photoacoustic computed tomography Chatni, M. R., Xia, J., Sohn, R., Maslov, K., Guo, Z., Zhang, Y., Wang, K., Xia, Y., Anastasio, M., Arbeit, J. and Wang, L. V., Jul 2012, In: Journal of biomedical optics. 17, 7, 076012.
- Whole-body ring-shaped confocal photoacoustic computed tomography of small animals in vivo Xia, J., Chatni, M. R., Maslov, K., Guo, Z., Wang, K., Anastasio, M. and Wang, L. V., May 2012, In: Journal of biomedical optics. 17, 5, 050506.
2011
- A layered media approach to photoacoustic tomography Schoonover, R. W. and Anastasio, M. A., May 5 2011, Photons Plus Ultrasound: Imaging and Sensing 2011. 78993P. (Progress in Biomedical Optics and Imaging - Proceedings of SPIE; vol. 7899).
- An imaging model incorporating ultrasonic transducer properties for three-dimensional optoacoustic tomography Wang, K., Ermilov, S. A., Su, R., Brecht, H. P., Oraevsky, A. A. and Anastasio, M. A., Feb 2011, In: IEEE Transactions on Medical Imaging. 30, 2, p. 203-214 12 p., 5560859.
- Compensation of shear waves in photoacoustic tomography with layered acoustic media Schoonover, R. W. and Anastasio, M. A., Oct 2011, In: Journal of the Optical Society of America A: Optics and Image Science, and Vision. 28, 10, p. 2091-2099 9 p.
- Estimate of effective singular values of a photoacoustic imaging system by noise characterization Roumeliotis, M. B., Anastasio, M. A. and Carson, J. J. L., May 5 2011, Photons Plus Ultrasound: Imaging and Sensing 2011. 78991U. (Progress in Biomedical Optics and Imaging - Proceedings of SPIE; vol. 7899).
- Feasibility study of complex wavefield retrieval in off-axis acoustic holography employing an acousto-optic sensor Rodríguez, G. L., Weber, J., Sandhu, J. S. and Anastasio, M. A., Dec 2011, In: Ultrasonics. 51, 8, p. 847-852 6 p.
- Image reconstruction in photoacoustic tomography involving layered acoustic media Schoonover, R. W. and Anastasio, M. A., Jun 2011, In: Journal of the Optical Society of America A: Optics and Image Science, and Vision. 28, 6, p. 1114-1120 7 p.
- Limited data image reconstruction in optoacoustic tomography by constrained, total variation minimization Wang, K., Sidky, E. Y., Anastasio, M. A., Oraevsky, A. A. and Pan, X., May 5 2011, Photons Plus Ultrasound: Imaging and Sensing 2011. 78993U. (Progress in Biomedical Optics and Imaging - Proceedings of SPIE; vol. 7899).
- Partial coherence and the transport of intensity and spectrum Zysk, A. M., Schoonover, R. W., Scott Carney, P. and Anastasio, M. A., 2011, Frontiers in Optics, FiO 2011. Optical Society of America (OSA), (Optics InfoBase Conference Papers).
- Potential for imaging engineered tissues with X-Ray phase contrast Appel, A., Anastasio, M. A. and Brey, E. M., Oct 1 2011, In: Tissue Engineering - Part B: Reviews. 17, 5, p. 321-330 10 p.
- Singular value decomposition analysis of a photoacoustic imaging system and 3D imaging at 0.7 FPS Roumeliotis, M. B., Stodilka, R. Z., Anastasio, M. A., Ng, E. and Carson, J. J. L., Jul 4 2011, In: Optics Express. 19, 14, p. 13405-13417 13 p.
- Task-based assessment of phase-contrast mammography Zysk, A. M. and Anastasio, M. A., 2011, Frontiers in Optics, FiO 2011. Optical Society of America (OSA), (Optics InfoBase Conference Papers).
2010
- 3D photoacoustic imaging Carson, J. J. L., Roumeliotis, M., Chaudhary, G., Stodilka, R. Z. and Anastasio, M. A., Nov 10 2010, Photonics North 2010. 775009. (Proceedings of SPIE - The International Society for Optical Engineering; vol. 7750).
- A layered media approach to photoacoustic tomography Schoonover, R. W. and Anastasio, M. A., Nov 8 2010, Image Reconstruction from Incomplete Data VI. 780003. (Proceedings of SPIE - The International Society for Optical Engineering; vol. 7800).
- Analysis of a photoacoustic imaging system by singular value decomposition Roumeliotis, M., Chaudhary, G., Anastasio, M., Stodilka, R., Immucci, A., Ng, E. and Carson, J. J. L., May 3 2010, Photons Plus Ultrasound: Imaging and Sensing 2010. 756432. (Progress in Biomedical Optics and Imaging - Proceedings of SPIE; vol. 7564).
- Analysis of a photoacoustic imaging system by the crosstalk matrix and singular value decomposition Roumeliotis, M., Stodilka, R. Z., Anastasio, M. A., Chaudhary, G., Al-Aabed, H., Ng, E., Immucci, A. and Carson, J. J. L., May 24 2010, In: Optics Express. 18, 11, p. 11406-11417 12 p.
- Analysis of ideal observer signal detectability in phase-contrast imaging employing linear shift-invariant optical systems Anastasio, M. A., Chou, C. Y., Zysk, A. M. and Brankov, J. G., Dec 2010, In: Journal of the Optical Society of America A: Optics and Image Science, and Vision. 27, 12, p. 2648-2659 12 p.
- Characterization of sparse-array detection photoacoustic tomography using the singular value decomposition Chaudhary, G., Roumeliotis, M., Ephrat, P., Stodilka, R., Carson, J. J. L. and Anastasio, M. A., May 3 2010, Photons Plus Ultrasound: Imaging and Sensing 2010. 756438. (Progress in Biomedical Optics and Imaging - Proceedings of SPIE; vol. 7564).
- Comparison of reconstruction algorithms for sparse-array detection photoacoustic tomography Chaudhary, G., Roumeliotis, M., Carson, J. J. L. and Anastasio, M. A., May 3 2010, Photons Plus Ultrasound: Imaging and Sensing 2010. 756434. (Progress in Biomedical Optics and Imaging - Proceedings of SPIE; vol. 7564).
- Compensation for transducer effects in optoacoustic tomography Wang, K., Anastasio, M. A., Ermilov, S. A., Brecht, H. P., Su, R. and Oraevsky, A. A., May 3 2010, Photons Plus Ultrasound: Imaging and Sensing 2010. 75640P. (Progress in Biomedical Optics and Imaging - Proceedings of SPIE; vol. 7564).
- Contributions to ideal observer SNRs in propagation-based X-ray phase-contrast imaging Anastasio, M. A., Chou, C. Y., Zysk, A. M. and Brankov, J. G., 2010, Medical Imaging 2010: Physics of Medical Imaging. PART 1 ed. 76220S. (Progress in Biomedical Optics and Imaging - Proceedings of SPIE; vol. 7622, no. PART 1).
- Image reconstruction exploiting object sparsity in boundary-enhanced X-ray phase-contrast tomography Sidky, E. Y., Anastasio, M. A. and Pan, X., May 10 2010, In: Optics Express. 18, 10, p. 10404-10422 19 p.
- Image reconstruction in photoacoustic tomography with variable speed of sound using a higher-order geometrical acoustics approximation Modgil, D., Anastasio, M. A. and Rivière, P. J. L., 2010, In: Journal of biomedical optics. 15, 2, 021308.
- Investigation of limited-view image reconstruction in optoacoustic tomography employing a priori structural information Huang, C., Oraevsky, A. A. and Anastasio, M. A., 2010, Image Reconstruction from Incomplete Data VI. 780004. (Proceedings of SPIE - The International Society for Optical Engineering; vol. 7800).
- Iterative image reconstruction for PROPELLER-MRI using the nonuniform fast fourier transform Tamhane, A. A., Anastasio, M. A., Gui, M. and Arfanakis, K., Jul 2010, In: Journal of Magnetic Resonance Imaging. 32, 1, p. 211-217 7 p.
- Noise texture and signal detectability in propagation-based x-ray phase-contrast tomography Chou, C. Y. and Anastasio, M. A., 2010, In: Medical Physics. 37, 1, p. 270-281 12 p.
- Poster — Thur Eve — 62: Analysis of a Photoacoustic Imaging System by Singular Value Decomposition Roumeliotis, M., Stodilka, R. Z., Anastasio, M. A. and Carson, J., Jul 2010, In: Medical Physics. 37, 7, p. 3899 1 p.
- Signal recovery and computational sensing and imaging: Introduction to the feature issue Testorf, M., Fiddy, M., Dogariu, A., Piestun, R., Matson, C. and Anastasio, M., Dec 1 2010, In: Applied Optics. 49, 34, p. SRC1-SRC2
- X-ray imaging of poly(ethylene glycol) hydrogels without contrast agents Brey, E. M., Appel, A., Chiu, Y. C., Zhong, Z., Cheng, M. H., Engel, H. and Anastasio, M. A., Dec 1 2010, In: Tissue Engineering - Part C: Methods. 16, 6, p. 1597-1600 4 p.
2009
- Analysis of the spectrum in phase-contrast mammography Zysk, A. M., Sisternes, L. D., Brankov, J. G., Xu, Q., Wernick, M. N. and Anastasio, M. A., Jun 15 2009, Medical Imaging 2009: Physics of Medical Imaging. 72584E. (Progress in Biomedical Optics and Imaging - Proceedings of SPIE; vol. 7258).
- Boundary reconstruction in limited-angle x-ray phase-contrast tomography Anastasio, M. A., Sidky, E. Y., Pan, X. and Chouc, C. Y., Jun 15 2009, Medical Imaging 2009: Physics of Medical Imaging. 725827. (Progress in Biomedical Optics and Imaging - Proceedings of SPIE; vol. 7258).
- Boundary-enhanced region-of-interest image reconstruction in propagation-based x-ray phase-contrast tomography Anastasio, M. A., Shi, D. and Pan, X., Dec 14 2009, In: Applied Physics Letters. 95, 24, 244101.
- Exploitation of symmetries for image reconstruction in linearized variable density diffraction tomography Shi, D. and Anastasio, M. A., Dec 29 2009, In: Journal of the Acoustical Society of America. 126, 6, p. 3095-3105 11 p.
- Image reconstruction in lens-based intensity diffraction tomography Huang, Y. and Anastasio, M. A., Jun 1 2009, In: Journal of the Optical Society of America A: Optics and Image Science, and Vision. 26, 6, p. 1335-1347 13 p.
- Image reconstruction in photoacoustic tomography with variable speed of sound using a higher-order geometrical acoustics approximation Modgil, D., Anastasio, M. A., Wang, K. and La Riviere, P. J., Jun 8 2009, In: Progress in Biomedical Optics and Imaging - Proceedings of SPIE. 7177, 71771A.
- Influence of imaging geometry on noise texture in quantitative in-line X-ray phase-contrast imaging Chou, C. Y. and Anastasio, M. A., Aug 17 2009, In: Optics Express. 17, 17, p. 14466-14480 15 p.
- Investigation of quantitative polychromatic X-ray phase-contrast tomography for tissue characterization Chou, C. Y., Zysk, A. M. and Anastasio, M. A., Jun 15 2009, Medical Imaging 2009: Physics of Medical Imaging. 72584B. (Progress in Biomedical Optics and Imaging - Proceedings of SPIE; vol. 7258).
- Multispectral intensity diffraction tomography: Single material objects with variable densities Anastasio, M. A., Xu, Q. and Shi, D., Feb 1 2009, In: Journal of the Optical Society of America A: Optics and Image Science, and Vision. 26, 2, p. 403-412 10 p.
- Photoacoustic image reconstruction in an attenuating medium using singular-value decomposition Modgil, D., Anastasio, M. A. and La Riviere, P. J., Jun 8 2009, In: Progress in Biomedical Optics and Imaging - Proceedings of SPIE. 7177, 71771B.
- Relationships between smooth-and small-phase conditions in X-ray phase-contrast imaging Shi, D. and Anastasio, M. A., Dec 2009, In: IEEE Transactions on Medical Imaging. 28, 12, p. 1969-1973 5 p., 5331297.
- Statistical properties of X-ray phase-contrast tomography Chou, C. Y. and Anastasio, M. A., 2009, Proceedings of the 31st Annual International Conference of the IEEE Engineering in Medicine and Biology Society: Engineering the Future of Biomedicine, EMBC 2009. IEEE Computer Society, p. 6648-6650 3 p. 5332868. (Proceedings of the 31st Annual International Conference of the IEEE Engineering in Medicine and Biology Society: Engineering the Future of Biomedicine, EMBC 2009).
2008
- Elucidation of 2D and 3D photoacoustic tomography Wang, K., Zhang, J. and Anastasio, M. A., Apr 23 2008, Photons Plus Ultrasound: Imaging and Sensing 2008: The Ninth Conference on Biomedical Thermoacoustics, Optoacoustics, and Acousto-optics. 68561E. (Progress in Biomedical Optics and Imaging - Proceedings of SPIE; vol. 6856).
- Improving limited-view reconstruction in photoacoustic tomography by incorporating a priori boundary information Anastasio, M. A., Wang, K., Zhang, J., Kruger, G. A., Reinecke, D. and Kruger, R. A., Apr 23 2008, Photons Plus Ultrasound: Imaging and Sensing 2008: The Ninth Conference on Biomedical Thermoacoustics, Optoacoustics, and Acousto-optics. 68561B. (Progress in Biomedical Optics and Imaging - Proceedings of SPIE; vol. 6856).
- Influence of imaging geometry on noise texture in X-ray in-line phase-contrast imaging Chou, C. Y. and Anastasio, M. A., May 14 2008, Medical Imaging 2008 - Physics of Medical Imaging. 69131Z. (Progress in Biomedical Optics and Imaging - Proceedings of SPIE; vol. 6913).
- Low density contrast agents for x-ray phase contrast imaging: The use of ambient air for x-ray angiography of excised murine liver tissue Laperle, C. M., Hamilton, T. J., Wintermeyer, P., Walker, E. J., Shi, D., Anastasio, M. A., Derdak, Z., Wands, J. R., Diebold, G. and Rose-Petruck, C., Dec 7 2008, In: Physics in medicine and biology. 53, 23, p. 6911-6923 13 p.
- Multi-bandwidth image reconstruction in photoacoustic tomography Chou, C. Y., Anastasio, M. A., Zhang, J., Ku, G., Yang, X. and Wang, L. V., Apr 23 2008, Photons Plus Ultrasound: Imaging and Sensing 2008: The Ninth Conference on Biomedical Thermoacoustics, Optoacoustics, and Acousto-optics. 68561G. (Progress in Biomedical Optics and Imaging - Proceedings of SPIE; vol. 6856).
- Multi-spectral intensity diffraction tomography Anastasio, M. A., Xu, Q. and Shi, D., Dec 17 2008, Image Reconstruction from Incomplete Data V. 707605. (Proceedings of SPIE - The International Society for Optical Engineering; vol. 7076).
- Noise properties of in-line X-ray imaging and tomography Chou, C. Y. and Anastasio, M. A., Nov 21 2008, Developments in X-Ray Tomography VI. 707813. (Proceedings of SPIE - The International Society for Optical Engineering; vol. 7078).
- Region-of-interest image reconstruction in X-ray differential phase-contrast tomography Anastasio, M. A., Xia, D. and Pan, X., May 14 2008, Medical Imaging 2008 - Physics of Medical Imaging. 691327. (Progress in Biomedical Optics and Imaging - Proceedings of SPIE; vol. 6913).
- Simultaneous reconstruction of speed-of-sound and optical absorption properties in photoacoustic tomography via a time-domain iterative algorithm Zhang, J., Wang, K., Yang, Y. and Anastasio, M. A., Apr 23 2008, Photons Plus Ultrasound: Imaging and Sensing 2008: The Ninth Conference on Biomedical Thermoacoustics, Optoacoustics, and Acousto-optics. 68561F. (Progress in Biomedical Optics and Imaging - Proceedings of SPIE; vol. 6856).
- Validity of a fully coherent field model for in-line x-ray phase imaging Zysk, A. M., Wernick, M. N. and Anastasio, M. A., 2008, Developments in X-Ray Tomography VI. 70781A. (Proceedings of SPIE - The International Society for Optical Engineering; vol. 7078).
- X-ray phase-contrast local tomography image reconstruction on pi-lines Anastasio, M. A., Shi, D. and Pan, X., Nov 21 2008, Developments in X-Ray Tomography VI. 707812. (Proceedings of SPIE - The International Society for Optical Engineering; vol. 7078).
2007
- An extended diffraction-enhanced imaging method for implementing multiple-image radiography Chou, C. Y., Anastasio, M. A., Brankov, J. G., Wernick, M. N., Brey, E. M., Connor, D. M. and Zhong, Z., Apr 7 2007, In: Physics in medicine and biology. 52, 7, p. 1923-1945 23 p., 011.
- Application of inverse source concepts to photoacoustic tomography Anastasio, M. A., Zhang, J., Modgil, D. and La Rivière, P. J., Dec 1 2007, In: Inverse Problems. 23, 6, p. S21-S35
- Comparison of iterative reconstruction approaches for photoacoustic tomography Zhang, J., Anastasio, M. A. and La Rivière, P. J., Apr 30 2007, Photons Plus Ultrasound: Imaging and Sensing 2007: The Eighth Conference on Biomedical Thermoacoustics, Optoacoustics, and Acousto-optics. 64370Y. (Progress in Biomedical Optics and Imaging - Proceedings of SPIE; vol. 6437).
- Image reconstruction in quantitative X-ray phase-contrast imaging employing multiple measurements Chou, C. Y., Huang, Y., Shi, D. and Anastasio, M. A., Aug 6 2007, In: Optics Express. 15, 16, p. 10002-10025 24 p.
- Intensity diffraction tomography with fixed detector plane Shi, D. and Anastasio, M. A., Oct 2007, In: Optical Engineering. 46, 10, 107003.
- Local cone-beam tomography image reconstruction on chords Anastasio, M. A., Zou, Y., Sidky, E. Y. and Pan, X., Jun 2007, In: Journal of the Optical Society of America A: Optics and Image Science, and Vision. 24, 6, p. 1569-1579 11 p.
- Perspectives in Fourier-based image reconstruction in photoacoustic tomography Anastasio, M. A., Zhang, J., Shi, D. and Pan, X., Apr 30 2007, Photons Plus Ultrasound: Imaging and Sensing 2007: The Eighth Conference on Biomedical Thermoacoustics, Optoacoustics, and Acousto-optics. 64371D. (Progress in Biomedical Optics and Imaging - Proceedings of SPIE; vol. 6437).
- Propagation based differential phase contrast imaging and tomography of murine tissue with a laser plasma x-ray source Laperle, C. M., Wintermeyer, P., Wands, J. R., Shi, D., Anastasio, M. A., Li, X., Ahr, B., Diebold, G. J. and Rose-Petruck, C., 2007, In: Applied Physics Letters. 91, 17, 173901.
- Region-of-interest imaging in differential phase-contrast tomography Anastasio, M. A. and Pan, X., Nov 1 2007, In: Optics Letters. 32, 21, p. 3167-3169 3 p.
- Statistically principled use of in-line measurements in intensity diffraction tomography Huang, Y. and Anastasio, M. A., Mar 2007, In: Journal of the Optical Society of America A: Optics and Image Science, and Vision. 24, 3, p. 626-642 17 p.
2006
- A comparison of a generalized DEI method with multiple-image radiography Chou, C. Y., Anastasio, M. A., Brankov, J. G. and Wernick, M. N., Nov 13 2006, Developments in X-Ray Tomography V. 631819. (Progress in Biomedical Optics and Imaging - Proceedings of SPIE; vol. 6318).
- A computed tomography implementation of multiple-image radiography Brankov, J. G., Wernick, M. N., Yang, Y., Li, J., Muehleman, C., Zhong, Z. and Anastasio, M. A., Feb 2006, In: Medical Physics. 33, 2, p. 278-289 12 p.
- A physical model of multiple-image radiography Khelashvili, G., Brankov, J. G., Chapman, D., Anastasio, M. A., Yang, Y., Zhong, Z. and Wernick, M. N., Jan 21 2006, In: Physics in medicine and biology. 51, 2, p. 221-236 16 p.
- Cone-beam local tomography image reconstruction on chords Anastasio, M. A., Sidky, E. Y., Zou, Y. and Pan, X., Jun 30 2006, Medical Imaging 2006: Physics of Medical Imaging. 614226. (Progress in Biomedical Optics and Imaging - Proceedings of SPIE; vol. 6142 II).
- Image reconstruction in optoacoustic tomography for dispersive acoustic media La Riviére, P. J., Zhang, J. and Anastasio, M. A., 2006, In: Optics Letters. 31, 6, p. 781-783 3 p.
- Image reconstruction in photoacoustic tomography with truncated cylindrical measurement apertures Anastasio, M. A. and Zhang, J., May 8 2006, Proceedings of SPIE - The International Society for Optical Engineering. 608610. (Proceedings of SPIE - The International Society for Optical Engineering; vol. 6086).
- Intensity diffraction tomography with a novel scanning protocol Shi, D. and Anastasio, M. A., Nov 9 2006, Image Reconstruction from Incomplete Data IV. 631607. (Proceedings of SPIE - The International Society for Optical Engineering; vol. 6316).
- Investigation of 3D microscopy using intensity diffraction tomography Anastasio, M. A., Huang, Y., Gbur, G. and Carney, P. S., 2006, Proceedings of SPIE - The International Society for Optical Engineering. 60900G. (Proceedings of SPIE - The International Society for Optical Engineering; vol. 6090).
- Multispectral intensity diffraction tomography reconstruction theory: Quasi-nondispersive objects Anastasio, M. A., Shi, D. and Gbur, G., Jun 2006, In: Journal of the Optical Society of America A: Optics and Image Science, and Vision. 23, 6, p. 1359-1368 10 p.
- Multi-spectral intensity diffraction tomography Anastasio, M. A., Shi, D. and Gbur, G., 2006, Frontiers in Optics, FiO 2006. Optical Society of America (OSA), (Optics InfoBase Conference Papers).
- Off-axis holographic tomography for diffracting scalar wavefields Shi, D. and Anastasio, M. A., Jan 2006, In: Physical Review E - Statistical, Nonlinear, and Soft Matter Physics. 73, 1, 016612.
- Progress in multiple-image radiography Wernick, M. N., Brankov, J. G., Chapman, D., Yang, Y., Khelashvili, G., Anastasio, M. A., Zhong, Z., Parham, C., Li, J. and Muehleman, C., Apr 17 2006, Computational Imaging IV - Proceedings of SPIE-IS and T Electronic Imaging. 60650W. (Proceedings of SPIE - The International Society for Optical Engineering; vol. 6065).
- Reconstruction of speed-of-sound and electromagnetic absorption distributions in photoacoustic tomography Zhang, J. and Anastasio, M. A., 2006, Proceedings of SPIE - The International Society for Optical Engineering. 608619. (Proceedings of SPIE - The International Society for Optical Engineering; vol. 6086).
- Statistically optimal image reconstruction for multi-detector intensity diffraction tomography Huang, Y. and Anastasio, M. A., Nov 9 2006, Image Reconstruction from Incomplete Data IV. 63160K. (Proceedings of SPIE - The International Society for Optical Engineering; vol. 6316).
- Three-dimensional image reconstruction in variable density acoustic tomography Anastasio, M. A. and Shi, D., Nov 9 2006, Image Reconstruction from Incomplete Data IV. 631604. (Proceedings of SPIE - The International Society for Optical Engineering; vol. 6316).
- Ultrasonic attenuation correction in optoacoustic tomography La Rivière, P. J., Zhang, J. and Anastasio, M. A., May 8 2006, Proceedings of SPIE - The International Society for Optical Engineering. 60861I. (Proceedings of SPIE - The International Society for Optical Engineering; vol. 6086).
2005
- Feasibility of half-data image reconstruction in 3-D reflectivity tomography with a spherical aperture Anastasio, M. A., Zhang, J., Sidky, E. Y., Zou, Y., Xia, D. and Pan, X., Sep 2005, In: IEEE Transactions on Medical Imaging. 24, 9, p. 1110-1112 3 p.
- Half-Time Image Reconstruction in Thermoacoustic Tomography Anastasio, M. A., Zhang, J., Pan, X., Zou, Y., Ku, G. and Wang, L. V., Feb 2005, In: IEEE transactions on medical imaging. 24, 2, p. 199-210 12 p.
- Image reconstruction in optoacoustic tomography accounting for frequency-dependent attenuation La Rivière, P. J., Jin, Z. and Anastasio, M. A., 2005, 2005 IEEE Nuclear Science Symposium Conference Record -Nuclear Science Symposium and Medical Imaging Conference. p. 1841-1845 5 p. 1596689. (IEEE Nuclear Science Symposium Conference Record; vol. 4).
- Image reconstruction in spherical-wave intensity diffraction tomography Anastasio, M. A., Shi, D., Huang, Y. and Gbur, G., Dec 2005, In: Journal of the Optical Society of America A: Optics and Image Science, and Vision. 22, 12, p. 2651-2661 11 p.
- Image reconstruction in thermoacoustic tomography with compensation for acoustic heterogeneities Anastasio, M. A., Zhang, J. and Pan, X., 2005, In: Progress in Biomedical Optics and Imaging - Proceedings of SPIE. 5750, p. 298-304 7 p., 32.
- Image reconstruction in variable density acoustic tomography Anastasio, M. A., Shi, D. and Deffieux, T., Sep 19 2005, In: Progress in Biomedical Optics and Imaging - Proceedings of SPIE. 5750, p. 326-331 6 p., 35.
- Intensity diffraction tomography using non-interferometric side-band holography Shi, D. and Anastasio, M. A., 2005, Frontiers in Optics, FiO 2005. Optical Society of America (OSA), (Optics InfoBase Conference Papers).
- k-space undersampling in PROPELLER imaging Arfanakis, K., Tamhane, A. A., Pipe, J. G. and Anastasio, M. A., Mar 2005, In: Magnetic Resonance in Medicine. 53, 3, p. 675-683 9 p.
- Mitigating artifacts via half-time reconstruction in thermoacoustic tomography Anastasio, M. A., Zhang, J., Pan, X., Ku, G. and Wang, L. V., 2005, In: Progress in Biomedical Optics and Imaging - Proceedings of SPIE. 5697, p. 263-270 8 p., 41.
- Phase-contrast tomography using tilted detector planes Anastasio, M. A. and Shi, D., Aug 25 2005, In: Progress in Biomedical Optics and Imaging - Proceedings of SPIE. 5745, II, p. 1266-1271 6 p., 149.
- Physical model of image formation in multiple-image radiography Brankov, J. G., Khelashvili, G., Chapman, D., Anastasio, M. A., Yang, Y., Zhong, Z. and Wernick, M. N., 2005, 2005 IEEE Nuclear Science Symposium Conference Record -Nuclear Science Symposium and Medical Imaging Conference. p. 2320-2322 3 p. 1596798. (IEEE Nuclear Science Symposium Conference Record; vol. 4).
- Reconstruction of refractive index discontinuities from truncated phase-contrast tomography projections Shi, D., Anastasio, M. A. and Pan, X., Jan 17 2005, In: Applied Physics Letters. 86, 3, p. 1-3 3 p., 034102.
- SU-FF-J-79: Local Tomography Using Adapted Feldkamp and Expectation Maximization Algorithms Zhang, J., Shi, D. and Anastasio, M., Jun 2005, In: Medical Physics. 32, 6, p. 1938 1 p.
- SU-FF-J-94: Comparison of Analytic and Iterative Algorithms for Image Reconstruction in Gated Megavoltage CT Zhang, J., Sillanpaa, J., Chang, J., Amols, H., Mageras, G. and Anastasio, M., Jun 2005, In: Medical Physics. 32, 6, p. 1941 1 p.
- Weighted expectation maximization reconstruction algorithms for thermoacoustic tomography Zhang, J., Anastasio, M. A., Pan, X. and Wang, L. V., Jun 2005, In: IEEE Transactions on Medical Imaging. 24, 6, p. 817-820 4 p.
- Weighted expectation maximization reconstruction algorithms with application to gated megavoltage tomography Zhang, J., Shi, D., Anastasio, M. A., Sillanpaa, J. and Chang, J., Nov 7 2005, In: Physics in medicine and biology. 50, 21, p. N299-N307
2004
- A preliminary study of multiple-image computed tomography Wernick, M. N., Brankov, J. G., Chapman, D., Yang, Y., Muehleman, C., Zhong, Z. and Anastasio, M. A., 2004, In: Proceedings of SPIE - The International Society for Optical Engineering. 5535, p. 369-379 11 p., 40.
- Analytic image reconstruction in local phase-contrast tomography Anastasio, M. A., Shi, D., De Carlo, F. and Pan, X., Jan 7 2004, In: Physics in medicine and biology. 49, 1, p. 121-144 24 p.
- Half-scan and single-plane intensity diffraction tomography for phase objects Shi, D., Anastasio, M. A., Huang, Y. and Gbur, G., Jun 21 2004, In: Physics in medicine and biology. 49, 12, p. 2733-2752 20 p.
- Half-time-based reflectivity tomography and its application to thermoacoustic tomography Anastasio, M. A., Pan, X., Zhang, J., Yu, Z., Xu, M. and Wang, L., 2004, In: Progress in Biomedical Optics and Imaging - Proceedings of SPIE. 5, 27, p. 80-86 7 p., 10.
- Image Reconstruction in Spherical-Wave Intensity Diffraction Tomography Anastasio, M. A., Shi, D., Huang, Y. and Gbur, G., 2004, Frontiers in Optics, FiO-2004. Optica Publishing Group (formerly OSA), FThP3. (Optics InfoBase Conference Papers).
- Intensity diffraction tomography for phase objects Shi, D., Anastasio, M. A., Huang, Y. and Gbur, G., Jul 5 2004, In: Proceedings of SPIE - The International Society for Optical Engineering. 5368, 2, p. 795-802 8 p.
- Multiple-image computed tomography Brankov, J. G., Wernick, M. N., Chapman, D., Zhong, Z., Muehleman, C., Li, J. and Anastasio, M. A., 2004, 2004 2nd IEEE International Symposium on Biomedical Imaging: Macro to Nano. p. 948-951 4 p. (2004 2nd IEEE International Symposium on Biomedical Imaging: Macro to Nano; vol. 1).
- Noise properties of intensity diffraction tomography Anastasio, M. A., Huang, Y., Shi, D. and Gbur, G., Jul 5 2004, In: Proceedings of SPIE - The International Society for Optical Engineering. 5368, 1, p. 272-280 9 p.
- On the relationship between intensity diffraction tomography and phase-contrast tomography Anastasio, M. A. and Shi, D., 2004, In: Proceedings of SPIE - The International Society for Optical Engineering. 5535, p. 361-368 8 p., 39.
- Phase-contrast tomography using incident spherical-waves Anastasio, M. A., Shi, D., Huang, Y. and Gbur, G., 2004, In: Proceedings of SPIE - The International Society for Optical Engineering. 5535, p. 724-732 9 p., 81.
- Quantitative boundary reconstruction from truncated phase-contrast tomographic projections Shi, D., Anastasio, M. A. and Pan, X., 2004, In: Proceedings of SPIE - The International Society for Optical Engineering. 5535, p. 310-317 8 p., 34.
2003
- A preliminary investigation of local tomography for megavoltage CT imaging Anastasio, M. A., Shi, D., Pan, X., Pelizzari, C. and Munro, P., Nov 2003, In: Medical Physics. 30, 11, p. 2969-2980 12 p.
- An improved reconstruction algorithm for 3-D diffraction tomography using spherical-wave sources Anastasio, M. A. and Pan, X., Apr 1 2003, In: IEEE Transactions on Biomedical Engineering. 50, 4, p. 517-521 5 p.
- Data redundancy and reduced-scan reconstruction in reflectivity tomography Pan, X., Zou, Y. and Anastasio, M. A., Jul 2003, In: IEEE Transactions on Image Processing. 12, 7, p. 784-795 12 p.
- Image reconstruction from reduced-scan data in ultrasonic reflectivity tomography Pan, X., Zou, Y., Anastasio, M. A. and Sidky, E., Sep 12 2003, In: Proceedings of SPIE - The International Society for Optical Engineering. 5035, p. 49-55 7 p.
- Investigation of Diffraction Tomography using Intensity Measurements Anastasio, M. A., Huang, Y., Shi, D. and Gbur, G., 2003, Frontiers in Optics, FiO 2003. Optica Publishing Group (formerly OSA), MQ5. (Optics InfoBase Conference Papers).
- Investigation of megavoltage local tomography for detecting setup errors in radiation therapy Shi, D., Anastasio, M. A., Pan, X., Pelizzari, C. and Munro, P., 2003, In: Proceedings of SPIE - The International Society for Optical Engineering. 5029, p. 176-182 7 p.
- Multiple-image radiography Wernick, M. N., Wirjadi, O., Chapman, D., Zhong, Z., Galatsanos, N. P., Yang, Y., Brankov, J. G., Oltulu, O., Anastasio, M. A. and Muehleman, C., Dec 7 2003, In: Physics in medicine and biology. 48, 23, p. 3875-3895 21 p.
- Phase-contrast tomography and the local tomography problem Anastasio, M. A., De Carlo, F. and Pan, X., Sep 15 2003, In: Proceedings of SPIE - The International Society for Optical Engineering. 5030 I, p. 120-126 7 p.
- Reconstructing the Location and Magnitude of Refractive Index Discontinuities from Truncated Phase-Contrast Tomographic Projections Anastasio, M. A., Shi, D., Huang, Y. and De Carlo, F., 2003, Frontiers in Optics, FiO 2003. Optica Publishing Group (formerly OSA), WM2. (Optics InfoBase Conference Papers).
2002
- A preliminary investigation of local cone-beam tomography for MV CT imaging of tumor volumes in radiation therapy Anastasio, M. A., Shi, D., Pan, X. and Pelizzari, C., 2002, In: Annual International Conference of the IEEE Engineering in Medicine and Biology - Proceedings. 2, p. 1186-1187 2 p.
- Data Truncation and the Exterior Reconstruction Problem in Reflection-Mode Tomography Zou, Y., Pan, X. and Anastasio, M. A., 2002, p. 726-730. 5 p.
- Image reconstruction of reflectivity from short scan data Pan, X., Zou, Y. and Anastasio, M. A., 2002, 2002 IEEE International Symposium on Biomedical Imaging, ISBI 2002 - Proceedings. IEEE Computer Society, p. 1027-1030 4 p. 1029439. (Proceedings - International Symposium on Biomedical Imaging; vol. 2002-January).
- Numerically robust minimal-scan reconstruction algorithms for diffraction tomography via Radon transform inversion Anastasio, M. A. and Pan, X., May 23 2002, In: International Journal of Imaging Systems and Technology. 12, 2, p. 84-91 8 p.
- On a limited-view reconstruction problem in diffraction tomography Pan, X. and Anastasio, M. A., Apr 2002, In: IEEE Transactions on Medical Imaging. 21, 4, p. 413-416 4 p.
- Reconstructing the Location and Magnitude of Refractive Index Discontinuities from Truncated Phase-Contrast Tomographic Projections Anastasio, M. A., Shi, D., Huang, Y. and De Carlo, F., 2003, Frontiers in Optics, FiO 2003. Optica Publishing Group (formerly OSA), WM2. (Optics InfoBase Conference Papers).
- Reflectivity tomography using temporally truncated data Anastasio, M. A., Zou, Y. and Pan, X., 2002, In: Annual International Conference of the IEEE Engineering in Medicine and Biology - Proceedings. 2, p. 921-922 2 p.
2001
- Comments on the filtered backprojection algorithm, range conditions, and the pseudoinverse solution Anastasio, M. A., Pan, X. and Clarkson, E., Jun 2001, In: IEEE Transactions on Medical Imaging. 20, 6, p. 539-542 4 p.
- Development and evaluation of minimal-scan reconstruction algorithms for diffraction tomography Anastasio, M. A. and Pan, X., 2001, In: Proceedings of SPIE - The International Society for Optical Engineering. 4322, 2, p. 860-866 7 p.
- Full- and minimal-scan reconstruction algorithms for fan-beam diffraction tomography Anastasio, M. A. and Pan, X., Jul 10 2001, In: Applied Optics. 40, 20, p. 3334-3345 12 p.
2000
- A new reconstruction approach for reflection mode diffraction tomography Anastasio, M. A. and Pan, X., Jul 2000, In: IEEE Transactions on Image Processing. 9, 7, p. 1262-1271 10 p.
- Computationally efficient and statistically robust image reconstruction in three-dimensional diffraction tomography Anastasio, M. A. and Pan, X., Mar 2000, In: Journal of the Optical Society of America A: Optics and Image Science, and Vision. 17, 3, p. 391-400 10 p.
- Minimal-scan reconstruction algorithms for fan-beam diffraction tomography and their analogy to halfscan fan-beam CT Pan, X. and Anastasio, M. A., 2000, p. 15/3-15/7.
- Multiobjective genetic optimization of diagnostic classifiers used in the computerized detection of mass lesions in mammography Kupinski, M. A., Anastasio, M. A. and Giger, M. L., 2000, In: Proceedings of SPIE - The International Society for Optical Engineering. 3979, p. I/-
1999
- A comparative study of image reconstructions in spect and ultrasonic diffraction tomography Pan, X., Anastasio, M. A. and Kao, C. M., 1999, In: IEEE Transactions on Nuclear Science. 46, 3 PART 2, p. 527-534 8 p.
- Comparative studies of image reconstructions in SPECT and diffraction tomography Pan, X., Anastasio, M. A. and Kao, C. M., 1999, IEEE Nuclear Science Symposium and Medical Imaging Conference. IEEE, p. 1673-1677 5 p. (IEEE Nuclear Science Symposium and Medical Imaging Conference; vol. 3).
- Fourier-based optimal recovery method for antialiasing interpolation Kao, C. M., Pan, X., La Riviere, P. and Anastasio, M. A., Dec 1999, In: Optical Engineering. 38, 12, p. 2041-2044 4 p.
- Interpolation method using signal recovery and discrete Fourier transform Kao, C. M., Pan, X., Anastasio, M. A. and La Riviere, P., 1999, IEEE Nuclear Science Symposium and Medical Imaging Conference. IEEE, p. 1387-1391 5 p. (IEEE Nuclear Science Symposium and Medical Imaging Conference; vol. 2).
- Investigation of the noise properties of a new class of reconstruction methods in diffraction tomography Anastasio, M. A. and Pan, X., 1999, In: International Journal of Imaging Systems and Technology. 10, 6, p. 437-445 9 p.
- Minimal-scan filtered backpropagation algorithms for diffraction tomography Pan, X. and Anastasio, M. A., Dec 1999, In: Journal of the Optical Society of America A: Optics and Image Science, and Vision. 16, 12, p. 2896-2903 8 p.
- Multidimensional smoothing using orthogonal expansions Anastasio, M. A., Pan, X. and Kao, C. M., Apr 1999, In: IEEE Signal Processing Letters. 6, 4, p. 91-94 4 p.
- Multiobjective approach to optimizing compterized detection schemes Anastasio, M. A., Kupinski, M. A., Nishikawa, R. M. and Giger, M. L., 1999, IEEE Nuclear Science Symposium and Medical Imaging Conference. IEEE, p. 1879-1883 5 p. (IEEE Nuclear Science Symposium and Medical Imaging Conference; vol. 3).
1998
- A genetic algorithm-based method for optimizing the performance of a computer-aided diagnosis scheme for detection of clustered microcalcifications in mammograms Anastasio, M. A., Yoshida, H., Nagel, R., Nishikawa, R. M. and Doi, K., Sep 1998, In: Medical Physics. 25, 9, p. 1613-1620 8 p.
- General technique for smoothing multi-dimensional datasets utilizing orthogonal expansions and lower dimensional smoothers Anastasio, M. A., Pan, X. and Kao, C. M., 1998, p. 718-721. 4 p.
- New classes of reconstruction methods in reflection mode diffraction tomography Anastasio, M. A., Kupinski, M. A. and Pan, X., 1998, In: Proceedings of the IEEE Ultrasonics Symposium. 1, p. 839-842 4 p.
- Noise propagation in diffraction tomography: comparison of conventional algorithms with a new reconstruction algorithm Anastasio, M. A., Kupinski, M. A. and Pan, X., Dec 1 1998, In: IEEE Transactions on Nuclear Science. 45, 4 PART 2, p. 2216-2223 8 p.
- Novel approach for a bias-free reduction of image variance in ultrasonic diffraction tomography Pan, X. and Anastasio, M., 1998, In: Proceedings of the IEEE Ultrasonics Symposium. 2, p. 1627-1630 4 p.
- Optimization and FROC analysis of rule-based detection schemes using a multiobjective approach Anastasio, M. A. and Kupinski, M. A., 1998, In: IEEE Transactions on Medical Imaging. 17, 6, p. 1089-1093 5 p.
1997
- Noise properties of reconstructed images in ultrasonic diffraction tomography Anastasio, M., Kupinski, M. and Pan, X., 1997, p. 1561-1565. 5 p.
-
2024
2023
2022
2021