Opposite Effects of Nonapeptide Antagonists on Paternal Behavior in the Teleost Fish Amphiprion Ocellaris
Ross DeAngelis, graduate research assistant in the Cellular and Molecular Foundations of Intelligent Behavior
ABSTRACT:
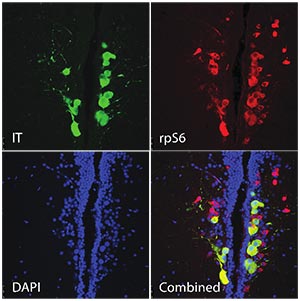
The nonapeptides isotocin (IT) and arginine vasotocin (AVT), along with their mammalian homologs oxytocin and arginine vasopressin, are well known regulators of social behaviors across vertebrate taxa. However, little is known about their involvement in paternal care. Here, we measured the effect of an IT and an AVT V1a receptor antagonist on paternal behaviors in the primarily paternal teleost Amphiprion ocellaris. We also measured effects of the IT receptor antagonist on aggression in dyadic contests between two non-reproductive fish to assess specificity of the effect on paternal behaviors. The IT receptor antagonist severely reduced paternal behaviors but had no effect on aggression, whereas the AVT V1a receptor antagonist increased paternal behaviors. Individual differences in levels of paternal behaviors (nips, fanning the eggs, and proportion of the time in the nest) were consistent across spawning cycles where no treatments were administered. These results support the idea that IT signaling is crucial for the expression of paternal behavior in A. ocellaris. Based on a previous study showing that the AVT V1a antagonist decreases aggression in dyadic contests, we hypothesize that the antagonist enhances paternal behavior indirectly by reducing vigilance and aggression, thereby alleviating effort directed towards other competing behaviors and allowing for the increased expression of paternal behaviors.
Imaging the Biomechanical Properties of Soft Tissues with Artificial Neural Networks
Cameron Hoerig, a Ph.D. student working with Michael Insana
ABSTRACT:
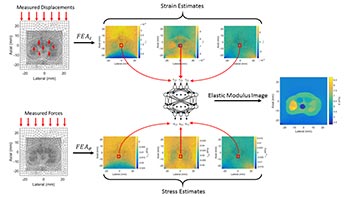
Elastography comprises a set of modalities that image the biomechanical properties of soft tissues for disease detection and diagnosis. Quasi-static ultrasound elastography, in particular, tracks sub-surface displacements resulting from an applied surface force. The local displacement information and measured surface loads may be used to compute a parametric summary of biomechanical properties; however, the inverse problem is ill-posed, limiting most techniques to estimating a single linear-elastic parameter. We are developing a new method of modeling mechanical properties that circumvents issues associated with directly solving the inverse problem. The Autoprogressive Method (AutoP) combines aspects of computational mechanics and machine learning to develop empirical models of soft tissue biomechanics without requiring initial assumptions of the underlying constitutive behavior. In AutoP, force-displacement measurements acquired during ultrasound imaging and knowledge of object geometry are used as input to finite element analyses to iteratively train artificial neural networks. The result is one or more neural network constitutive models that describe the mechanical behavior governing the measured force-displacement data, from which mechanical parameters can be estimated.
AutoP has been used to successfully create models for linear-elastic gelatin gels and has promising preliminary results for biological media. Moving forward, the method will be adapted to build models of non-linear and viscoelastic properties as well as incorporate spatial information to remove the need for prior knowledge of object shape.
Global Optimal Nonlinear Adaptive Control through Domain Linearization
Luke Wendt, from Stephen Levinson’s Language Acquisition and Robotics Group
ABSTRACT:
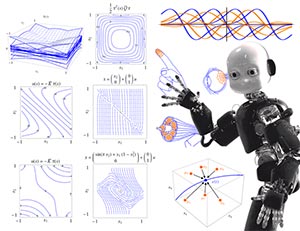
Multivariate nonlinear dynamic systems defined on a finite domain can be made into computationally equivalent high dimensional linear systems through a generalizable reinterpretation of the state. This new form of linearization uses a classical neural inspired basis and may provide insight into analogues biological forms of intelligence. These tools can characterize adaptive control of the surrounding environment as well as co-operative behavior with agents that have limited communication and influence. The mathematics will be primarily restricted to continuous time deterministic systems with state space representation, but these results can be extended to discrete time stochastic systems.
By transforming any nonlinear system into its linear form, all the tools of optimal linear control theory can be applied globally over the domain of linearization. In particular, control and estimation problems involving under-actuated under-measured nonlinear systems with complex nonlinear reward can now be solved with linear theory. The controllable, uncontrollable, stabilizable, observable, unobservable, and detectable subspaces can all be described in a meaningful way. The final solutions can be expressed in computationally efficient low dimensional highly nonlinear form. This is a new approach from traditional methods like gain scheduling and feedback linearization.